Order Now
- Home
- About Us
-
Services
-
Assignment Writing
-
Academic Writing Services
- HND Assignment Help
- SPSS Assignment Help
- College Assignment Help
- Writing Assignment for University
- Urgent Assignment Help
- Architecture Assignment Help
- Total Assignment Help
- All Assignment Help
- My Assignment Help
- Student Assignment Help
- Instant Assignment Help
- Cheap Assignment Help
- Global Assignment Help
- Write My Assignment
- Do My Assignment
- Solve My Assignment
- Make My Assignment
- Pay for Assignment Help
-
Management
- Management Assignment Help
- Business Management Assignment Help
- Financial Management Assignment Help
- Project Management Assignment Help
- Supply Chain Management Assignment Help
- Operations Management Assignment Help
- Risk Management Assignment Help
- Strategic Management Assignment Help
- Logistics Management Assignment Help
- Global Business Strategy Assignment Help
- Consumer Behavior Assignment Help
- MBA Assignment Help
- Portfolio Management Assignment Help
- Change Management Assignment Help
- Hospitality Management Assignment Help
- Healthcare Management Assignment Help
- Investment Management Assignment Help
- Market Analysis Assignment Help
- Corporate Strategy Assignment Help
- Conflict Management Assignment Help
- Marketing Management Assignment Help
- Strategic Marketing Assignment Help
- CRM Assignment Help
- Marketing Research Assignment Help
- Human Resource Assignment Help
- Business Assignment Help
- Business Development Assignment Help
- Business Statistics Assignment Help
- Business Ethics Assignment Help
- 4p of Marketing Assignment Help
- Pricing Strategy Assignment Help
- Nursing
-
Finance
- Finance Assignment Help
- Do My Finance Assignment For Me
- Financial Accounting Assignment Help
- Behavioral Finance Assignment Help
- Finance Planning Assignment Help
- Personal Finance Assignment Help
- Financial Services Assignment Help
- Forex Assignment Help
- Financial Statement Analysis Assignment Help
- Capital Budgeting Assignment Help
- Financial Reporting Assignment Help
- International Finance Assignment Help
- Business Finance Assignment Help
- Corporate Finance Assignment Help
-
Accounting
- Accounting Assignment Help
- Managerial Accounting Assignment Help
- Taxation Accounting Assignment Help
- Perdisco Assignment Help
- Solve My Accounting Paper
- Business Accounting Assignment Help
- Cost Accounting Assignment Help
- Taxation Assignment Help
- Activity Based Accounting Assignment Help
- Tax Accounting Assignment Help
- Financial Accounting Theory Assignment Help
-
Computer Science and IT
- Operating System Assignment Help
- Data mining Assignment Help
- Robotics Assignment Help
- Computer Network Assignment Help
- Database Assignment Help
- IT Management Assignment Help
- Network Topology Assignment Help
- Data Structure Assignment Help
- Business Intelligence Assignment Help
- Data Flow Diagram Assignment Help
- UML Diagram Assignment Help
- R Studio Assignment Help
-
Law
- Law Assignment Help
- Business Law Assignment Help
- Contract Law Assignment Help
- Tort Law Assignment Help
- Social Media Law Assignment Help
- Criminal Law Assignment Help
- Employment Law Assignment Help
- Taxation Law Assignment Help
- Commercial Law Assignment Help
- Constitutional Law Assignment Help
- Corporate Governance Law Assignment Help
- Environmental Law Assignment Help
- Criminology Assignment Help
- Company Law Assignment Help
- Human Rights Law Assignment Help
- Evidence Law Assignment Help
- Administrative Law Assignment Help
- Enterprise Law Assignment Help
- Migration Law Assignment Help
- Communication Law Assignment Help
- Law and Ethics Assignment Help
- Consumer Law Assignment Help
- Science
- Biology
- Engineering
-
Humanities
- Humanities Assignment Help
- Sociology Assignment Help
- Philosophy Assignment Help
- English Assignment Help
- Geography Assignment Help
- Agroecology Assignment Help
- Psychology Assignment Help
- Social Science Assignment Help
- Public Relations Assignment Help
- Political Science Assignment Help
- Mass Communication Assignment Help
- History Assignment Help
- Cookery Assignment Help
- Auditing
- Mathematics
-
Economics
- Economics Assignment Help
- Managerial Economics Assignment Help
- Econometrics Assignment Help
- Microeconomics Assignment Help
- Business Economics Assignment Help
- Marketing Plan Assignment Help
- Demand Supply Assignment Help
- Comparative Analysis Assignment Help
- Health Economics Assignment Help
- Macroeconomics Assignment Help
- Political Economics Assignment Help
- International Economics Assignments Help
-
Academic Writing Services
-
Essay Writing
- Essay Help
- Essay Writing Help
- Essay Help Online
- Online Custom Essay Help
- Descriptive Essay Help
- Help With MBA Essays
- Essay Writing Service
- Essay Writer For Australia
- Essay Outline Help
- illustration Essay Help
- Response Essay Writing Help
- Professional Essay Writers
- Custom Essay Help
- English Essay Writing Help
- Essay Homework Help
- Literature Essay Help
- Scholarship Essay Help
- Research Essay Help
- History Essay Help
- MBA Essay Help
- Plagiarism Free Essays
- Writing Essay Papers
- Write My Essay Help
- Need Help Writing Essay
- Help Writing Scholarship Essay
- Help Writing a Narrative Essay
- Best Essay Writing Service Canada
-
Dissertation
- Biology Dissertation Help
- Academic Dissertation Help
- Nursing Dissertation Help
- Dissertation Help Online
- MATLAB Dissertation Help
- Doctoral Dissertation Help
- Geography Dissertation Help
- Architecture Dissertation Help
- Statistics Dissertation Help
- Sociology Dissertation Help
- English Dissertation Help
- Law Dissertation Help
- Dissertation Proofreading Services
- Cheap Dissertation Help
- Dissertation Writing Help
- Marketing Dissertation Help
- Programming
-
Case Study
- Write Case Study For Me
- Business Law Case Study Help
- Civil Law Case Study Help
- Marketing Case Study Help
- Nursing Case Study Help
- Case Study Writing Services
- History Case Study help
- Amazon Case Study Help
- Apple Case Study Help
- Case Study Assignment Help
- ZARA Case Study Assignment Help
- IKEA Case Study Assignment Help
- Zappos Case Study Assignment Help
- Tesla Case Study Assignment Help
- Flipkart Case Study Assignment Help
- Contract Law Case Study Assignments Help
- Business Ethics Case Study Assignment Help
- Nike SWOT Analysis Case Study Assignment Help
- Coursework
- Thesis Writing
- CDR
- Research
-
Assignment Writing
-
Resources
- Referencing Guidelines
-
Universities
-
Australia
- Asia Pacific International College Assignment Help
- Macquarie University Assignment Help
- Rhodes College Assignment Help
- APIC University Assignment Help
- Torrens University Assignment Help
- Kaplan University Assignment Help
- Holmes University Assignment Help
- Griffith University Assignment Help
- VIT University Assignment Help
- CQ University Assignment Help
-
Australia
- Experts
- Free Sample
- Testimonial
DATA4000 Introduction to Business Analytics Assignment Sample
Assignment Brief
Word Count - 2000 Words (+/-10%)
Weighting - 30 %
Total Marks - 30
Submission: via Turnitin
Due Date - Monday Week 5, 23:55pm AEDT
Your Task
Complete Parts A to C below by the due date. Consider the rubric at the end of the assignment guidance on structure and content.
Assessment Description
• You are to read case studies provided and answer questions in relation to the content, analytics theory and potential analytics professionals required for solving the business problems at hand.
• Learning outcomes 1 and 2 are addressed.
Assessment Instructions for assignment help
Part A: Case Study Analysis (700 words, 10 marks)
Instructions: Read the following two case studies. For each case study, briefly describe:
a) The industry to which analytics has been applied
b) A potential and meaningful business problem to be solved
c) The type of analytics used, and how it was used to address that potential and meaningful business problem
d) The main challenge(s) of using this type of analytics to achieve your business objective (from part b)
e) Recommendations regarding how to be assist stakeholders with adapting these applications
for their business.
1. Artificial Intelligence in Germany: Reinventing Engines of Growth
https://www.bernardmarr.com/default.asp?contentID=2141
2. GE Power: Big Data, Machine learning and ‘The Internet of Energy’
https://www.bernardmarr.com/default.asp?contentID=1226
Part B: The Role of Analytics in Solving Business Problems (500 words, 8 marks)
Instructions: Describe two different types of analytics (from Workshop 1) and evaluate how each could be used as part of a solution to a business problem with reference to ONE real-world case study of your own choosing. You will need to conduct independent research and consult resources provided in the subject.
Part C: Developing and Sourcing Analytics Capabilities (800 words, 12 marks)
Instructions: You are the Chief Analytics Officer for a large multinational corporation in the communications sector with operations that span South East Asia and Latin America. The organization is undergoing significant transformations; it is scaling back operations in existing low revenue segments and ramping up investments in next generation products and services - 5G, cloud computing and Software as a Service (SaaS). The business is keen to develop its data and analytics capabilities. This includes using technology for product innovation and for developing a large contingent of knowledge workers.
To prepare management for these changes, you have been asked review Accenture’s report
(see link below) and publish a short report of your own that addresses the following key points:
1. How do we best ingrain analytics into our decision-making processes?
2. How do we organize and coordinate analytics capabilities across the organization?
3. How should we source, train and deploy analytics talent?
To help you draft this report, you should review the following working paper from Accenture:
https://www.accenture.com/us-en/~/media/accenture/conversion-
assets/dotcom/documents/global/pdf/industries_2/accenture-building-analytics-driven-
organization.pdf
The report is prepared for senior management and the board of directors. It must reflect the needs of your organization and the sector you operate in (communications).
Solution
Part A: Case Study Analysis
A. The industry in which analytics has been used
The case studies concern themselves in two different aspects of analytics. The case study named 'Artificial Intelligence in Germany: Reinventing Engines of Growth' deals with the artificial intelligence analytic. Another case study named GE Power: Big Data, Machine learning, and 'The Internet of Energy' is related to block chain analytic. In the case study concerning artificial intelligence, several industries are introduced to use artificial intelligence analytics. Germany introduced AI in its traditional businesses at first. Company Otto, a giant E-commerce company, uses AI for analytics implementation (Marr 2020a). Another company Zalando, an E-tailer organization, uses AI for the performance management of the machines. Germany's largest railway infrastructure provider company Siemens AG uses AI for its railway infrastructure analysis (Marr 2020a).
Moreover, the automobile companies such as BMW and Daimler also use AI technology in their autonomous vehicles. Allianz Insurance company utilizes AI analytics (Marr 2020a). Also, Braun uses AI in its digital toothbrush making, where the AI technology will help to implement proper ways to brush the teeth. Media Conglomerate Bertelsmann hosted AI for solving data and AI challenges in business (Marr 2020a). Another case study related to block chain analytics targets the health care industry to solve the issues in operational management (Marr 2020b). MedRec uses block chains to improve electronic medical records, allowing patients' information to be accessed securely (Marr 2020b).
B. The potential and meaningful business problem to be solved
The possible and potential issues can be solved through strengthening AI research, using a competitive market to drive innovation for encouraging SMEs to use AI in business structure. The e-commerce company Otto has the problem of product returns in its online commerce market (Marr 2020a). Zalando has been facing the issue of fraudulence in the e-tailer system. Siemens AG wants to avoid the issues of delay in trains arrival. To promote reliability and accuracy is the target. BMW and Daimler are facing issues in traditional man-driven vehicles. For operational efficacy improvement, Allianz Insurance uses AI (Marr 2020a). Bruan targets healthy habit which is reasonably related to clinical activities to prevent teeth problems. Bertelsmann has faced challenges in business. The case study concerning block chain analytics corresponds to various issues related to health information. The case study shows around 140 million patient's records have been breached between 2015 to 2016 as per the report of the Protenus Breach Barometer (Marr 2020b). IT architecture is struggling to secure systems. It becomes an issue to generate and record data in disparate systems through any human. Medicare fraud is also an issue. They reduce the counterfeit drug application costs high to pharmaceutical companies, which have created a loss of $200 million (Marr 2020b). The issues such as waste of time, duplication in process, money, confusion, and life-threatening are related to MediRec.
C. The type of analytics used and how it was used to address that potential and meaningful business problem
Artificial intelligence and blockchains are the analytics used. Germany introduced AI to solve the operational issues related to products and services (Marr 2020a). The government wants to become one of the leaders of global technology. For that, business organisations in Germany focus on quality over quantity as the competition relating to AI technology is high in the global market. AI can benefit the citizens in Germany to experience a high level of IT security. For that, AI technology must be implemented legally and ethically. Otto uses algorithm processes in online activities to decrease product returns (Marr 2020a). Zalando uses AI to prevent fraud in the e-tailer system. The automobile vehicle companies BMW and Daimler use AI to produce autonomous vehicles to solve the prevalent issue. Bertelsmann uses AI to develop intelligent solutions to avoid possible issues in business (Marr 2020a). The teams focused on various tasks such as voice-controlled search functions, automated podcasts, listening recommendations. Higher privacy and security provision are agendas to use block chain analytics (Marr 2020b). Block chain infrastructure can be a possible solution to minimize the risk of patient record breaching. Block chain helps to disparate the systems for future treatment. Reducing the counterfeit drug application costs high to pharmaceutical companies can be managed through block chain (Marr 2020b). To deal with the issues such as waste of time, duplication in process, money, confusion, and life-threatening issue, MediRec applied block chain (Marr 2020b).
D. The main challenges in using the analytics to achieve business objective
The challenges concerning utilizing analytics such as artificial intelligence and block chain to achieve the business goal can be customer dissatisfaction, driver downtime, and reducing maintenance costs. Moreover, the issues regarding data security and privacy are a significant concern in both AI and Block chain. Artificial intelligence helps in securing the system from fraudulent transactions, but the barrier is to handle the system. For that, organisations need to hire IT experts, which will make the organisations bear additional costs. Kroger, while using artificial intelligence to Restock Krogers, can face significant challenges. Among them, data security while the transaction is a significant issue.
Furthermore, record tracking, sharing, assigning, and linking can be faced while using the blockchain analytic. For instance, Meta Capital has been facing challenges while implementing the blockchain technology in partnership channel with ConsenSys Config. Blockchain is used to reduce delay in banking sectors, but the cost of blockchain operation is high.
E. Recommendations regarding stakeholders’ assistance
Managing the business objective through using analytics needs to assist the stakeholders about application adaptation.
• Training regarding analytics such as artificial intelligence and blockchain is a must for the stakeholders.
• The stakeholders need to engage themselves with IT experts to know the system management through analytics.
• Discussion regarding the aim or objective with the stakeholders through meetings and conferences can be practical.
• The stakeholders need to know about the transformations after implementing the analytics in the system.
• Awareness must be created regarding the need for innovation to achieve organizational success.
• Training regarding privacy and security maintenance must be given to the stakeholders while operating through analytics.
Part B: The Role of Analytics in solving Business Problem
Analytics has immense contribution to business due to its ability in identifying problems and providing new opportunities to solve these problems. Analytics not only help in making business decisions but also in identifying opportunities to engage a more significant number of customers. In the case of Artificial intelligence, it is used to make a market survey and identify customer requirements by trend analysis. The critical business decisions regarding the product that needs to be more highlighted or updated and suggesting the products according to customer needs are made through artificial intelligence. Business analytics is also helpful in dealing with logistics issues where several shipping companies use analytics to keep track of their fleet. The sensor devices will help diagnose the problem in parts of the ship, which will reduce maintenance cost, driver downtime and customer dissatisfaction. Predictions related to internal problems within the organization can be solved through predictive analytics that helps in identifying the issues, predict what will happen in future through past data and make suggestions or recommendations accordingly (Michigan state university online 2020). Artificial Intelligence is making its mark in business organizations for dealing with issues like data security and fraudulent transactions for giving more safe and secured solutions in the business.
Nowadays, many online transactions occur through mobile phones using the internet, where personal data is shared constantly. Kroger using Artificial Intelligence in their initiative Restock Kroger where the shoppers are allowed to scan groceries through their smartphone using AI. They have also planned for an autonomous vehicle for delivery in partnership with Nuro, Silicon Valley Company. They are also planning to have robotics in their operations to design an automated warehouse where robots using Machine learning algorithm can select products for delivery from warehouses. Using smart shelves technology, customers will be given suggestions based on their choice and price demands. Machine learning will help in the deployment of several models without human interventions (Bernardmarr 2020). The data transfer process is speedy, and companies face a massive challenge in dealing with the security problem. Using Artificial Intelligence, the process of transaction scanning for potential fraud detection takes place at a fast rate, making the system more secure. Block chain technology is used in supply chain management where asset recording, tracking, sharing, assigning and linking makes it efficient, giving more transparency and better protection at every step. For example, Mata Capital has partnered with ConsenSys Config to leverage its assets by introducing security tokens using block chain technology. It has helped in better dealing with customer requirements, cost befits, more security in transactions, and business expansion to a new class of investors (Consensys 2020). block chain is used in several banking sectors to assist in cross-border transactions and reduce delay. The processing taking place in real-time ensures more security and restricts any securing them from any modifications. Data security is also a significant issue in the business where the personal data of customers and sensitive information of organizations are stored in large databases, which is prone to any security breach and malicious attack. Blockchain-enabled security systems are more effective as any attack has done to blockchain-based data storage will be fatal, and there is no way of tampering or stealing the data (Goodfirms 2021).
Part C: Developing and Sourcing Analytics Capabilities
1. As a chief analytics officer in the communication sector, it can be asserted that including analytics in the decision-making process is significant, which helps to manage the service changes. For infusing analytics in the decision-making process, CPG leaders addressed the business issues initially and then defined the data (Hernandez, Berkey & Bhattacharya 2013). After this process, they reengineer decisions for result analysis and insight. CPG focuses upon rare skill development for ingraining analytics into decision-making processes. The cross-functional process is also effective as it helps the business produces a greater return to analytics capabilities. It also enhances the price, promotion, and assortment efficiency. CPG enhances speed and end-to-end process assessment in decision making, which helps to infuse analytics in business decision making. In the decision-making process, changes for enhanced performance need fact-based discussion and action in brand marketing, field sales, supply chain, and sales planning. Reengineering the decision-making process in business will help to function the analytics-driven process in business. Analytics promote quicker decision-making abilities. Also, the organisations have to analyze their ability to manage the reengineering process. For example, P&G company uses data-driven culture and innovative tools like the business sphere to infuse analytics in the decision-making process (Hernandez, Berkey & Bhattacharya 2013).
Conducting an analytics diagnosis process will help to identify the type of insight needed and also determines who and when will develop the insight so that timely delivery can be possible. Organizational changes that enhance the value of Analytics and visibility are also required. Consistent and deliberate typing strategies and tactics will generate insights from Analytics. Analytics services aim to reach enterprise business goals which involve the prioritizing process.
2. Managing transformation in business due to technological inclusion needs to have organization and coordination analytics capabilities across the organization. The closest positioning to decision making and delivering the most value includes analytics insight development. Organisations construct and allocate resources based on business needs and maturity. Managing supply and demand for analytics services across the business is crucial. The fundamental organizational issues such as sponsorship, leadership, funding, and governance are addressed to extract value from analytics (Hernandez, Berkey & Bhattacharya 2013). For maintaining the balance of demand and supply, sponsorship helps to create benefits of analytics to whip up energy and confidence. The view of enterprise helps in making required decisions that benefit the organization. The ultimate objective of the sponsor is to accelerate adoption and buy-in within the organization. Sponsors need to be an astute leader in breaking the cultural barriers to disseminate data widely.
The leadership in organisations creates a considerable impact on the coordination of analytics capabilities. Analytics leaders encourage vision regarding the capabilities of them the organization in holding people accountable for the result. The leader has to build analytics capability in culture and improves the decision-making process. Funding comes from various sources or functions. In proportion, function puts on priority upon Analytics. The funding promotes the capabilities of analytics. It increases simultaneously with the proportion of analytics in the organization. Funding in organisations would signify the strategic value of Analytics which generates, preserving the point of migrating to a pay-to-play model (Hernandez, Berkey & Bhattacharya 2013). The pay-to-play model creates the ability for the functional executives for integrating Analytics insight development into business decisions. Due to the increment in adoption, resources become more appropriate.
Governance involves the ownership of analytics and the capability to manage demand and supply. Different roles and responsibilities correlate with the governance structure. The initial considerations involve the maturity of analytics capabilities, priorities of the organization, and the need to balance demand and supply. The centralizing model helps to grow demands for analytics. The Federated model also works when there is high demand in analytics, including the SWAT team to manage complex cross-functional decisions. (Hernandez, Berkey & Bhattacharya 2013) Governance reduced duplication KPIs establishment is done for the central and dispersed teams. Finally, Analytics resources provide capability development opportunities. Stakeholders' engagement in the analytics process is also significant for supply and demand management. Management of demand helps to identify, prioritise and service the highest value opportunities.
3. Sourcing, training, and deploying analytics talent includes several aspects regarding talent management. The seven components used to shape an appropriate operating model are sponsorship and governance, organizational structure and talent management, data of insights, capability development, Insight-driven decision, outcome measurements, and information and data management. Organisations for understanding the talent needs will use analytical skills. In that case, an analyst is needed who will understand the distribution network. CPG companies tend to have talent in descriptive Analytics (Hernandez, Berkey & Bhattacharya 2013). The company needs to generate predictive and prescriptive insights. Talent sourcing is another aspect that can be done in a variety of ways. The public-private partnership is effective in resourcing as universities like MIT invest in data science degree programs for talent sourcing. Even Google, Microsoft, and Amazon are supporting the programs related to Analytics for talent sourcing. Alternative arrangements can provide a dedicated capacity of analytics talent (Hernandez, Berkey & Bhattacharya 2013). Flexibility and capacity promote lower costs in comparison to the internal hiring process. Capacity development involves business skills to remain relevant with strategic management. It also includes executive-level skills. Ability to manipulate, find and interpret and manage the data help in developing capacity. Talent management requires a new approach beyond the standard career improvement. For instance, CGP needs to work hard to create a path that will retain the analytics talent.
References
Bernardmarr 2020, Kroger: How This U.S. Retail Giant Is Using AI And Robots To Prepare For The 4th Industrial Revolution, viewed 19 April 2021, <https://bernardmarr.com/default.asp?contentID=1537>.
Consensys 2020, Codefi Case Study Download: Mata Capital, viewed 19 April 2021, <https://pages.consensys.net/codefi-mata-capital-case-study>.
Goodfirms 2021, ‘Top 10 Problems that Blockchain Solves’, viewed 19 April 2021, <https://www.goodfirms.co/blog/problems-blockchain-solves#:~:text=One%20thing%20is%20certain%20now,charity%2C%20voting%2C%20and%20crowdfunding>.
Hernandez, J, Berkey, B & Bhattacharya, R 2013, Building-analytics-driven-organization. viewed 19 April 2021, <https://www.accenture.com/us-en/~/media/accenture/conversion-assets/dotcom/documents/global/pdf/industries_2/accenture-building-analytics-driven-organization.pdf>.
Marr, B 2020a, Artificial Intelligence In Germany, viewed 19 April 2021, <https://www.bernardmarr.com/default.asp?contentID=2141>.
Marr, B 2020b, This Is Why Blockchains Will Transform Healthcare, viewed 19 April 2021, <https://www.bernardmarr.com/default.asp?contentID=1226>.
Michigan state university online 2020, How Business Analytics Can Help Your Business, viewed 19 April 2021, <https://www.michiganstateuniversityonline.com/resources/business-analytics/how-business-analytics-can-help-your-business/>.
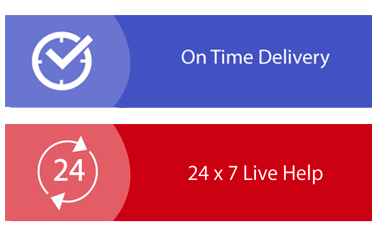
Download Samples PDF
Related Sample
- FIN2SEV Investment Securities Assignment
- BST720 Leadership Work and Organization Assignment
- EDUC8731 Motivation Cognition and Metacognition Assignment
- PUBHLTH7113 Environmental and Occupation Health Assignment
- MGT603 Systems Thinking Report
- COIT20261 Network Routing and Switching Term Assignment
- CSE2HUM Ethical Issue on Current Cyber Context Assignment
- PBHL20001 Understanding Public Health Assignment
- BUACC5930 Accounting and Finance Assignment
- MET 414 Applied Epidemiology Assignment
- Effect of Australian Engineering Ethics on Professionalism and Society
- ACCT20080 Governance and Ethics Assignment
- Impact of US China War On The Chinese Economy and Its Long Term Implications
- BULAW5914 Commercial Law Assignment
- Strategic Management Assignment
- ENEM28001 FEA for Engineering Design Report
- COIT20251 Knowledge Audit for Business Analysis Report
- TSM11105 International Tourism Assignment
- BUS3002 Business School Industrial Experience Assignment
- TCHR5003 Principles and Practices in Early Childhood Assignment
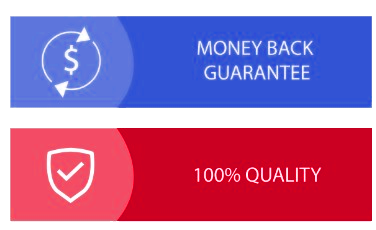
Assignment Services
-
Assignment Writing
-
Academic Writing Services
- HND Assignment Help
- SPSS Assignment Help
- College Assignment Help
- Writing Assignment for University
- Urgent Assignment Help
- Architecture Assignment Help
- Total Assignment Help
- All Assignment Help
- My Assignment Help
- Student Assignment Help
- Instant Assignment Help
- Cheap Assignment Help
- Global Assignment Help
- Write My Assignment
- Do My Assignment
- Solve My Assignment
- Make My Assignment
- Pay for Assignment Help
-
Management
- Management Assignment Help
- Business Management Assignment Help
- Financial Management Assignment Help
- Project Management Assignment Help
- Supply Chain Management Assignment Help
- Operations Management Assignment Help
- Risk Management Assignment Help
- Strategic Management Assignment Help
- Logistics Management Assignment Help
- Global Business Strategy Assignment Help
- Consumer Behavior Assignment Help
- MBA Assignment Help
- Portfolio Management Assignment Help
- Change Management Assignment Help
- Hospitality Management Assignment Help
- Healthcare Management Assignment Help
- Investment Management Assignment Help
- Market Analysis Assignment Help
- Corporate Strategy Assignment Help
- Conflict Management Assignment Help
- Marketing Management Assignment Help
- Strategic Marketing Assignment Help
- CRM Assignment Help
- Marketing Research Assignment Help
- Human Resource Assignment Help
- Business Assignment Help
- Business Development Assignment Help
- Business Statistics Assignment Help
- Business Ethics Assignment Help
- 4p of Marketing Assignment Help
- Pricing Strategy Assignment Help
- Nursing
-
Finance
- Finance Assignment Help
- Do My Finance Assignment For Me
- Financial Accounting Assignment Help
- Behavioral Finance Assignment Help
- Finance Planning Assignment Help
- Personal Finance Assignment Help
- Financial Services Assignment Help
- Forex Assignment Help
- Financial Statement Analysis Assignment Help
- Capital Budgeting Assignment Help
- Financial Reporting Assignment Help
- International Finance Assignment Help
- Business Finance Assignment Help
- Corporate Finance Assignment Help
-
Accounting
- Accounting Assignment Help
- Managerial Accounting Assignment Help
- Taxation Accounting Assignment Help
- Perdisco Assignment Help
- Solve My Accounting Paper
- Business Accounting Assignment Help
- Cost Accounting Assignment Help
- Taxation Assignment Help
- Activity Based Accounting Assignment Help
- Tax Accounting Assignment Help
- Financial Accounting Theory Assignment Help
-
Computer Science and IT
- Operating System Assignment Help
- Data mining Assignment Help
- Robotics Assignment Help
- Computer Network Assignment Help
- Database Assignment Help
- IT Management Assignment Help
- Network Topology Assignment Help
- Data Structure Assignment Help
- Business Intelligence Assignment Help
- Data Flow Diagram Assignment Help
- UML Diagram Assignment Help
- R Studio Assignment Help
-
Law
- Law Assignment Help
- Business Law Assignment Help
- Contract Law Assignment Help
- Tort Law Assignment Help
- Social Media Law Assignment Help
- Criminal Law Assignment Help
- Employment Law Assignment Help
- Taxation Law Assignment Help
- Commercial Law Assignment Help
- Constitutional Law Assignment Help
- Corporate Governance Law Assignment Help
- Environmental Law Assignment Help
- Criminology Assignment Help
- Company Law Assignment Help
- Human Rights Law Assignment Help
- Evidence Law Assignment Help
- Administrative Law Assignment Help
- Enterprise Law Assignment Help
- Migration Law Assignment Help
- Communication Law Assignment Help
- Law and Ethics Assignment Help
- Consumer Law Assignment Help
- Science
- Biology
- Engineering
-
Humanities
- Humanities Assignment Help
- Sociology Assignment Help
- Philosophy Assignment Help
- English Assignment Help
- Geography Assignment Help
- Agroecology Assignment Help
- Psychology Assignment Help
- Social Science Assignment Help
- Public Relations Assignment Help
- Political Science Assignment Help
- Mass Communication Assignment Help
- History Assignment Help
- Cookery Assignment Help
- Auditing
- Mathematics
-
Economics
- Economics Assignment Help
- Managerial Economics Assignment Help
- Econometrics Assignment Help
- Microeconomics Assignment Help
- Business Economics Assignment Help
- Marketing Plan Assignment Help
- Demand Supply Assignment Help
- Comparative Analysis Assignment Help
- Health Economics Assignment Help
- Macroeconomics Assignment Help
- Political Economics Assignment Help
- International Economics Assignments Help
-
Academic Writing Services
-
Essay Writing
- Essay Help
- Essay Writing Help
- Essay Help Online
- Online Custom Essay Help
- Descriptive Essay Help
- Help With MBA Essays
- Essay Writing Service
- Essay Writer For Australia
- Essay Outline Help
- illustration Essay Help
- Response Essay Writing Help
- Professional Essay Writers
- Custom Essay Help
- English Essay Writing Help
- Essay Homework Help
- Literature Essay Help
- Scholarship Essay Help
- Research Essay Help
- History Essay Help
- MBA Essay Help
- Plagiarism Free Essays
- Writing Essay Papers
- Write My Essay Help
- Need Help Writing Essay
- Help Writing Scholarship Essay
- Help Writing a Narrative Essay
- Best Essay Writing Service Canada
-
Dissertation
- Biology Dissertation Help
- Academic Dissertation Help
- Nursing Dissertation Help
- Dissertation Help Online
- MATLAB Dissertation Help
- Doctoral Dissertation Help
- Geography Dissertation Help
- Architecture Dissertation Help
- Statistics Dissertation Help
- Sociology Dissertation Help
- English Dissertation Help
- Law Dissertation Help
- Dissertation Proofreading Services
- Cheap Dissertation Help
- Dissertation Writing Help
- Marketing Dissertation Help
- Programming
-
Case Study
- Write Case Study For Me
- Business Law Case Study Help
- Civil Law Case Study Help
- Marketing Case Study Help
- Nursing Case Study Help
- Case Study Writing Services
- History Case Study help
- Amazon Case Study Help
- Apple Case Study Help
- Case Study Assignment Help
- ZARA Case Study Assignment Help
- IKEA Case Study Assignment Help
- Zappos Case Study Assignment Help
- Tesla Case Study Assignment Help
- Flipkart Case Study Assignment Help
- Contract Law Case Study Assignments Help
- Business Ethics Case Study Assignment Help
- Nike SWOT Analysis Case Study Assignment Help
- Coursework
- Thesis Writing
- CDR
- Research