Order Now
- Home
- About Us
-
Services
-
Assignment Writing
-
Academic Writing Services
- Urgent Assignment Help
- Writing Assignment for University
- College Assignment Help
- SPSS Assignment Help
- HND Assignment Help
- Architecture Assignment Help
- Total Assignment Help
- All Assignment Help
- My Assignment Help
- Student Assignment Help
- Instant Assignment Help
- Cheap Assignment Help
- Global Assignment Help
- Write My Assignment
- Do My Assignment
- Solve My Assignment
- Make My Assignment
- Pay for Assignment Help
-
Management
- Financial Management Assignment Help
- Business Management Assignment Help
- Management Assignment Help
- Project Management Assignment Help
- Supply Chain Management Assignment Help
- Operations Management Assignment Help
- Risk Management Assignment Help
- Strategic Management Assignment Help
- Logistics Management Assignment Help
- Global Business Strategy Assignment Help
- Consumer Behavior Assignment Help
- MBA Assignment Help
- Portfolio Management Assignment Help
- Change Management Assignment Help
- Hospitality Management Assignment Help
- Healthcare Management Assignment Help
- Investment Management Assignment Help
- Market Analysis Assignment Help
- Corporate Strategy Assignment Help
- Conflict Management Assignment Help
- Marketing Management Assignment Help
- Strategic Marketing Assignment Help
- CRM Assignment Help
- Marketing Research Assignment Help
- Human Resource Assignment Help
- Business Assignment Help
- Business Development Assignment Help
- Business Statistics Assignment Help
- Business Ethics Assignment Help
- 4p of Marketing Assignment Help
- Pricing Strategy Assignment Help
- Nursing
-
Finance
- Finance Assignment Help
- Do My Finance Assignment For Me
- Financial Accounting Assignment Help
- Behavioral Finance Assignment Help
- Finance Planning Assignment Help
- Personal Finance Assignment Help
- Financial Services Assignment Help
- Forex Assignment Help
- Financial Statement Analysis Assignment Help
- Capital Budgeting Assignment Help
- Financial Reporting Assignment Help
- International Finance Assignment Help
- Business Finance Assignment Help
- Corporate Finance Assignment Help
-
Accounting
- Accounting Assignment Help
- Managerial Accounting Assignment Help
- Taxation Accounting Assignment Help
- Perdisco Assignment Help
- Solve My Accounting Paper
- Business Accounting Assignment Help
- Cost Accounting Assignment Help
- Taxation Assignment Help
- Activity Based Accounting Assignment Help
- Tax Accounting Assignment Help
- Financial Accounting Theory Assignment Help
-
Computer Science and IT
- Robotics Assignment Help
- Operating System Assignment Help
- Data mining Assignment Help
- Computer Network Assignment Help
- Database Assignment Help
- IT Management Assignment Help
- Network Topology Assignment Help
- Data Structure Assignment Help
- Business Intelligence Assignment Help
- Data Flow Diagram Assignment Help
- UML Diagram Assignment Help
- R Studio Assignment Help
-
Law
- Law Assignment Help
- Business Law Assignment Help
- Contract Law Assignment Help
- Tort Law Assignment Help
- Social Media Law Assignment Help
- Criminal Law Assignment Help
- Employment Law Assignment Help
- Taxation Law Assignment Help
- Commercial Law Assignment Help
- Constitutional Law Assignment Help
- Corporate Governance Law Assignment Help
- Environmental Law Assignment Help
- Criminology Assignment Help
- Company Law Assignment Help
- Human Rights Law Assignment Help
- Evidence Law Assignment Help
- Administrative Law Assignment Help
- Enterprise Law Assignment Help
- Migration Law Assignment Help
- Communication Law Assignment Help
- Law and Ethics Assignment Help
- Consumer Law Assignment Help
- Science
- Biology
- Engineering
-
Humanities
- Humanities Assignment Help
- Sociology Assignment Help
- Philosophy Assignment Help
- English Assignment Help
- Geography Assignment Help
- Agroecology Assignment Help
- Psychology Assignment Help
- Social Science Assignment Help
- Public Relations Assignment Help
- Political Science Assignment Help
- Mass Communication Assignment Help
- History Assignment Help
- Cookery Assignment Help
- Auditing
- Mathematics
-
Economics
- Economics Assignment Help
- Managerial Economics Assignment Help
- Econometrics Assignment Help
- Microeconomics Assignment Help
- Business Economics Assignment Help
- Marketing Plan Assignment Help
- Demand Supply Assignment Help
- Comparative Analysis Assignment Help
- Health Economics Assignment Help
- Macroeconomics Assignment Help
- Political Economics Assignment Help
- International Economics Assignments Help
-
Academic Writing Services
-
Essay Writing
- Essay Help
- Essay Writing Help
- Essay Help Online
- Online Custom Essay Help
- Descriptive Essay Help
- Help With MBA Essays
- Essay Writing Service
- Essay Writer For Australia
- Essay Outline Help
- illustration Essay Help
- Response Essay Writing Help
- Professional Essay Writers
- Custom Essay Help
- English Essay Writing Help
- Essay Homework Help
- Literature Essay Help
- Scholarship Essay Help
- Research Essay Help
- History Essay Help
- MBA Essay Help
- Plagiarism Free Essays
- Writing Essay Papers
- Write My Essay Help
- Need Help Writing Essay
- Help Writing Scholarship Essay
- Help Writing a Narrative Essay
- Best Essay Writing Service Canada
-
Dissertation
- Biology Dissertation Help
- Academic Dissertation Help
- Nursing Dissertation Help
- Dissertation Help Online
- MATLAB Dissertation Help
- Doctoral Dissertation Help
- Geography Dissertation Help
- Architecture Dissertation Help
- Statistics Dissertation Help
- Sociology Dissertation Help
- English Dissertation Help
- Law Dissertation Help
- Dissertation Proofreading Services
- Cheap Dissertation Help
- Dissertation Writing Help
- Marketing Dissertation Help
- Programming
-
Case Study
- Write Case Study For Me
- Business Law Case Study Help
- Civil Law Case Study Help
- Marketing Case Study Help
- Nursing Case Study Help
- Case Study Writing Services
- History Case Study help
- Amazon Case Study Help
- Apple Case Study Help
- Case Study Assignment Help
- ZARA Case Study Assignment Help
- IKEA Case Study Assignment Help
- Zappos Case Study Assignment Help
- Tesla Case Study Assignment Help
- Flipkart Case Study Assignment Help
- Contract Law Case Study Assignments Help
- Business Ethics Case Study Assignment Help
- Nike SWOT Analysis Case Study Assignment Help
- Coursework
- Thesis Writing
- CDR
- Research
-
Assignment Writing
-
Resources
- Referencing Guidelines
-
Universities
-
Australia
- Asia Pacific International College Assignment Help
- Macquarie University Assignment Help
- Rhodes College Assignment Help
- APIC University Assignment Help
- Torrens University Assignment Help
- Kaplan University Assignment Help
- Holmes University Assignment Help
- Griffith University Assignment Help
- VIT University Assignment Help
- CQ University Assignment Help
-
Australia
- Experts
- Free Sample
- Testimonial
MBA673 Business Analytics Lifecycle Report 3 Sample
Your Task
Creating an analytics project roadmap
• This report is to be done individually and submitted via Turnitin on Tuesday week 13 23:55 AEDT.
• Covers LO1 to LO4
Assessment Instructions
Section 1: [ 500 words, 10 marks]
a. Describe the different phases of the business analytics lifecycle that you have learnt about week by week during this trimester, i.e. asking meaningful business questions, data discovery and preparation, forecasting, etc.
Section 2: [ 750 words, 15 marks] Consider the ‘garments_worker_productivity.csv’ dataset that you used in assessment 2 and answer the following:
b. Articulate a few business questions in relation to that data set.
c. What type of data cleaning and preparation would you possibly apply to this data?
d. What type of analytics (or models) did you apply to the data and could you potentially apply to this data in the future?
e. Compare correlation and causation. Consider variables such as incentive and actual_productivity. What methods could you use to find evidence for causality in this case, i.e. incentives cause higher productivity.
f. By considering the task you did in assessment 2 as a project, describe how you could extend or automate it.
g. Section 3: [ 250 words, 5 marks] Create a cycle or flow chart to represent the project road map and summarise each component of the cycle/flow chart.
Solution
a. In order to use data effectively to generate actionable insights and make wise business decisions, each stage is essential. The many phases I have researched week by week are described as follows:
1. Understanding the business challenge and Formulating Meaningful Business Questions: This is the first step in the process. University Assignment Help, As it establishes the framework for the entire analytics procedure, this phase is essential. Analysts identify the main areas of concern and create quantifiable targets to direct the following steps in collaboration with stakeholders.
2. Data Discovery and Preparation: Following the identification of the business questions comes the phase of data discovery and preparation. Finding and gathering the pertinent data from numerous internal and external sources is what this stage comprises. To assure the quality and suitability of the data for analysis, analysts must clean, validate, and convert it. Dealing with missing values, outliers, and inconsistent data may also be part of this procedure.
3. Exploratory Data Analysis: To comprehend the dataset more thoroughly, exploratory data analysis (EDA) is carried out after data preparation. Data patterns, correlations, and trends are found using EDA techniques including data visualization, summary statistics, and correlation analysis. EDA aids analysts in producing hypotheses for additional research and identifying prospective variables of interest.
4. Statistical Modelling and Analysis: During this stage, statistical modeling methods are used to analyze the data and provide answers to the queries posed by the company. To create models that can explain and forecast the phenomenon under research, many statistical techniques such as regression analysis, time series analysis, classification algorithms, and others are used. The models are verified with the proper assessment criteria and improved as required.
5. Predictive analytics and forecasting: A key component of business analytics is forecasting, which is speculating on potential future trends, demands, and consequences. Key variables of interest are forecast using time series analysis, machine learning algorithms, and other predictive modeling methods. Businesses may foresee changes in the market, allocate resources efficiently, and take aggressive action during this time.
6. Reporting and visualization: After the analysis is complete, it is important to properly inform the stakeholders of the results. Reporting entails delivering the findings in an understandable and succinct manner, frequently making use of visualizations such graphs, charts, and dashboards. These visual representations make it easier to understand complicated information and to make decisions.
7. Making decisions and putting them into practise: The last stage of the business analytics lifecycle is using the insights gained from the analysis to guide decisions. Decision-makers analyze the results, weigh possible risks and advantages, and choose the best course of action. Monitoring and assessment occur concurrently with decision-making in order to gauge the effectiveness of the selected course of action.
An iterative and collaborative approach must be maintained throughout the business analytics lifecycle. The relevance and efficacy of the insights produced are ensured through feedback from stakeholders, ongoing model review, and improvement of analytic tools. To ensure ethical and reliable analytics practices, regulatory compliance, data protection, and other factors must be incorporated across the whole lifespan.
b. Based on the 'garments_worker_productivity.csv' dataset, here are a few business questions that can be explored:
1. How does the authority's intended productivity compare to the actual productivity of the workforce?
2. What connection exists between productivity and overtime? Does working extra hours result in increased or decreased productivity?
3. Is there a connection between the volume of style modifications and output? Do frequent changes in style affect employees' productivity?
4. Does the money given to employees have a beneficial impact on productivity? Does the quantity of the incentive correlate with actual productivity?
5. How does productivity differ depending on the department and team size? Do certain teams or departments routinely produce more than others?
6. Does the actual productivity obtained depend on the standard minute value (SMV) allotted for each task?
c. To make sure the "garments_worker_productivity.csv" dataset is trustworthy and appropriate for analysis, there are a number of critical procedures that may be taken during data cleaning and preparation.
1. The first step is to locate and deal with any missing values in the dataset. Missing values can be filled up with appropriate values based on the nature of the variable or, if required, the relevant rows or columns can be removed. This makes sure that a whole dataset is used for the study.
2. Outliers and other data anomalies should be controlled. Making a decision on how to manage outliers is crucial since they can have a major impact on the analysis's findings. If extreme findings are found to be inaccurate or deceptive, they can be removed. or they can be transformed or replaced with more appropriate values.
3. The next step is to make sure that each variable's data type is valid and that the appropriate format has been allocated. Dates, for instance, need to be translated to the suitable date format, and categorical variables need to be given the right data type. This guarantees correctness and consistency in subsequent analyses.
4. If necessary, approaches for normalizing or scaling variables can be used. When using some methods that are sensitive to discrepancies in magnitude, normalization guarantees that variables are on a comparable scale.
5. To analyze patterns at a deeper level, data aggregation may also be taken into consideration. To determine average productivity or other aggregated metrics, the data must be aggregated by team, department, or other pertinent characteristics. This offers a wider perspective and aids in seeing patterns or trends that might not be obvious at the level of a single record.
6. Validating data is also very important. To find any differences or errors, it is necessary to compare the data with domain knowledge or other trustworthy sources. It makes sure the data is of a high caliber and increases the validity of the analysis's findings.
The dataset is made more trustworthy, consistent, and analytically ready by carrying out extensive data cleaning and preparation. By doing this, the possibility of bias is reduced and the accuracy and reliability of the insights and conclusions reached through the analytics process are guaranteed.
d. One might use numerous analytics models and methodologies on the dataset from the prior assessment. Some of the potential strategies include:
1. Descriptive statistics: Descriptive statistics give us quick access to metrics that provide a spotlight on the structure, dispersion, and central tendency of the variables in the dataset. A quantitative summary of the data is provided by measures like mean, median, mode, standard deviation, and range. These statistics serve as a starting point for additional study by allowing us to spot patterns, trends, and variances in the variables.
2. Regression analysis: Examine the link between different independent variables (such as overtime, incentives, and SMV and the dependent variable (actual productivity) using regression models in order to discover key variables that have a substantial impact on production.
3. Time Series Analytics: Analyze productivity trends over time, taking into account seasonality, trends, and possible patterns, using time series models like ARIMA or SARIMA.
4. Classification Models: Build classification models that forecast levels of productivity based on other factors, such as department, team, or style changes.
5. Clustering analysis: Utilize clustering analysis to find teams or groups of employees who have comparable productivity trends.
6. Predictive analytics: To forecast future events, predictive analytics uses historical data and a variety of statistical modeling approaches. Predictive analytics may be used to anticipate productivity levels in the context of the dataset depending on elements like department, team, incentive amount, and other variables. Predictive models may be built using machine learning techniques such as decision trees, random forests, or gradient boosting.
7. Prescriptive Analytics: By offering suggestions for the best courses of action to take in order to achieve desired results, prescriptive analytics goes beyond predictive analytics. Prescriptive analytics can assist in determining the most productive course of action for the dataset. For instance, it can recommend the best distribution of resources across teams or departments, identify the best incentive system, or offer suggestions for process changes to boost efficiency.
8. Forecasting models: Create forecasting models to project future productivity using data from the past and other pertinent factors.
e. The concepts of correlation and causation are different. A statistical metric known as correlation shows the degree to which two variables are related, whereas causation suggests that one variable causes the other.
Some techniques that can be used to determine whether there is a causal relationship between elements like incentives and actual production are:
1. Conduct a controlled experiment in which one group of employees is given incentives while the other is not. If the group receiving incentives continuously outperforms the other group, causality can be deduced by comparing the productivity levels between the two groups.
2. In randomized controlled trials (RCTs), workers are divided into two groups, one of which is given incentives and the other is not. Causal linkages can be established by contrasting the results between the two groups.
3. Use statistical approaches like propensity score matching or instrumental variable analysis to account for confounding variables and establish a causal association as part of a causal inference process.
It's crucial to remember that proving causation necessitates careful study design, accounting for potential confounding variables, and reproducing findings in order to guarantee dependability.
f. To extend or automate the project from Assessment 2, several steps can be taken:
1. Include other data sources: Include data from extra relevant sources, such as staff performance measurements, client feedback, or market trends, to get a more in-depth insight of what influences productivity.
2. Construct real-time dashboards: Make dynamic dashboards that continuously update with fresh data to give stakeholders access to current information on productivity levels, trends, and performance indicators.
3. Using predictive models Create artificial intelligence models that can forecast future productivity based on a variety of variables. This may make it possible to plan resources and make preemptive decisions.
4. Establishing methods for detecting anomalies Utilize machine learning tools to spot odd production trends or anomalies so that timely action can be taken to prevent disruptions.
5. Automate the preparation and cleansing of data: Create automated workflows or scripts to perform routine data preparation and cleaning chores, such as missing value imputation, outlier identification, and data type conversions.
6. Utilize machine learning algorithms to deploy recommendation systems that make suggestions for process enhancements, resource allocation, or incentive structures based on past trends and industry best practices.
7. Put automatic reporting into practice: Create automatic reports that highlight performance data, provide actionable advice for various stakeholders, and summarize significant results.
8. Keep an eye on and assess: Follow up on any comments you receive, keep an eye on how well the solutions you've adopted are working, and keep refining your models and procedures to get better results over time.
Organizations can gain from more timely and accurate insights, simpler procedures, and data-driven decision-making by extending and automating the project, which will ultimately increase productivity and performance.
G.
Summary:
1. Problem Identification & Defining Objectives: During this phase, the project's business problem is located and its specific project objectives are specified. In order to comprehend their requirements and concerns, stakeholders must work together.
2. Data collection, Preparation & Exploratory Data Analysis: This stage focuses on gathering pertinent data from multiple sources, both internal and external. The gathered data is subsequently checked, converted, and made ready for analysis. The prepared data is then utilized for exploratory data analysis to uncover new information and comprehend the properties of the dataset. Patterns and relationships are found using visualizations, summary statistics, and correlation analysis.
3. Statistical Modelling and Analytics: To analyze the data and respond to particular business problems, statistical modeling techniques are used. These techniques include regression analysis, time series analysis, and classification algorithms.
4. Predictive Analytics, Reporting & Visualization: Developing models and methods to predict future trends, requests, and results based on previous data is the goal of the phase.. It supports future productivity forecasting, risk detection, and resource allocation optimization. Clear and succinct reports, visualizations, and dashboards are used to convey the analysis' conclusions. In this stage, the results are presented to the stakeholders in a clear and usable form.
5. Decision Making and Implementation: During this phase, analysis-derived insights are applied to make well-informed judgements. Decision-makers analyze the results, weigh the risks and rewards, and take action according to the suggested solutions.
6. Monitoring and Evaluation: During this stage, the decisions that have been put into action are monitored and their effects are assessed. In order to evaluate the success of the solutions and pinpoint opportunities for development, key performance indicators are monitored.
References:
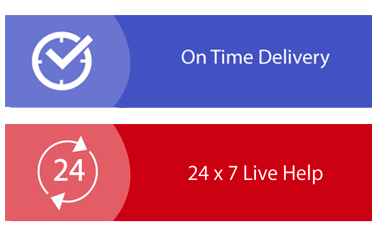
Download Samples PDF
Related Sample
- ISYS1003 Cybersecurity Management
- PUBH6012 Capstone B Applied Research Project in Public Health Assignment
- CAP203 Care of The Person with an Acute Illness Case Study
- NSG3101 Rehabilitation and Community Cares Assignment
- Novel Protein Acherons Distribution Using Fluorescence Microscopy Assignment
- MBA401 People Culture and Contemporary Leadership
- MBA401 People Culture and Contemporary Leadership Assignment
- Effect of Australian Engineering Ethics on Professionalism and Society
- BFA704 Work Placement Assignment
- Information Security Assignment
- DASE201 Data Security Assignment
- MIS500 Foundations of Information Systems Assignment
- MITS5003 Wireless Networks and Communication Case Study
- COIT20250 Emerging Technologies in E business Assignment
- MANU2123 External Project Proposal Assignment
- MGT602 Business Decision Analytics Report
- Dynamic Leadership Assignment
- LAW500 Business Law Assignment
- HAS108 Health Assessment
- An Essay on Accounts Receivable Assignment
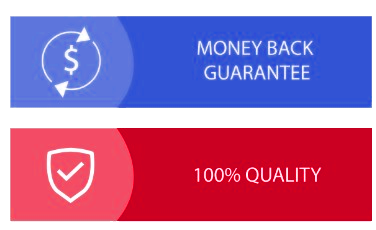
Assignment Services
-
Assignment Writing
-
Academic Writing Services
- Urgent Assignment Help
- Writing Assignment for University
- College Assignment Help
- SPSS Assignment Help
- HND Assignment Help
- Architecture Assignment Help
- Total Assignment Help
- All Assignment Help
- My Assignment Help
- Student Assignment Help
- Instant Assignment Help
- Cheap Assignment Help
- Global Assignment Help
- Write My Assignment
- Do My Assignment
- Solve My Assignment
- Make My Assignment
- Pay for Assignment Help
-
Management
- Financial Management Assignment Help
- Business Management Assignment Help
- Management Assignment Help
- Project Management Assignment Help
- Supply Chain Management Assignment Help
- Operations Management Assignment Help
- Risk Management Assignment Help
- Strategic Management Assignment Help
- Logistics Management Assignment Help
- Global Business Strategy Assignment Help
- Consumer Behavior Assignment Help
- MBA Assignment Help
- Portfolio Management Assignment Help
- Change Management Assignment Help
- Hospitality Management Assignment Help
- Healthcare Management Assignment Help
- Investment Management Assignment Help
- Market Analysis Assignment Help
- Corporate Strategy Assignment Help
- Conflict Management Assignment Help
- Marketing Management Assignment Help
- Strategic Marketing Assignment Help
- CRM Assignment Help
- Marketing Research Assignment Help
- Human Resource Assignment Help
- Business Assignment Help
- Business Development Assignment Help
- Business Statistics Assignment Help
- Business Ethics Assignment Help
- 4p of Marketing Assignment Help
- Pricing Strategy Assignment Help
- Nursing
-
Finance
- Finance Assignment Help
- Do My Finance Assignment For Me
- Financial Accounting Assignment Help
- Behavioral Finance Assignment Help
- Finance Planning Assignment Help
- Personal Finance Assignment Help
- Financial Services Assignment Help
- Forex Assignment Help
- Financial Statement Analysis Assignment Help
- Capital Budgeting Assignment Help
- Financial Reporting Assignment Help
- International Finance Assignment Help
- Business Finance Assignment Help
- Corporate Finance Assignment Help
-
Accounting
- Accounting Assignment Help
- Managerial Accounting Assignment Help
- Taxation Accounting Assignment Help
- Perdisco Assignment Help
- Solve My Accounting Paper
- Business Accounting Assignment Help
- Cost Accounting Assignment Help
- Taxation Assignment Help
- Activity Based Accounting Assignment Help
- Tax Accounting Assignment Help
- Financial Accounting Theory Assignment Help
-
Computer Science and IT
- Robotics Assignment Help
- Operating System Assignment Help
- Data mining Assignment Help
- Computer Network Assignment Help
- Database Assignment Help
- IT Management Assignment Help
- Network Topology Assignment Help
- Data Structure Assignment Help
- Business Intelligence Assignment Help
- Data Flow Diagram Assignment Help
- UML Diagram Assignment Help
- R Studio Assignment Help
-
Law
- Law Assignment Help
- Business Law Assignment Help
- Contract Law Assignment Help
- Tort Law Assignment Help
- Social Media Law Assignment Help
- Criminal Law Assignment Help
- Employment Law Assignment Help
- Taxation Law Assignment Help
- Commercial Law Assignment Help
- Constitutional Law Assignment Help
- Corporate Governance Law Assignment Help
- Environmental Law Assignment Help
- Criminology Assignment Help
- Company Law Assignment Help
- Human Rights Law Assignment Help
- Evidence Law Assignment Help
- Administrative Law Assignment Help
- Enterprise Law Assignment Help
- Migration Law Assignment Help
- Communication Law Assignment Help
- Law and Ethics Assignment Help
- Consumer Law Assignment Help
- Science
- Biology
- Engineering
-
Humanities
- Humanities Assignment Help
- Sociology Assignment Help
- Philosophy Assignment Help
- English Assignment Help
- Geography Assignment Help
- Agroecology Assignment Help
- Psychology Assignment Help
- Social Science Assignment Help
- Public Relations Assignment Help
- Political Science Assignment Help
- Mass Communication Assignment Help
- History Assignment Help
- Cookery Assignment Help
- Auditing
- Mathematics
-
Economics
- Economics Assignment Help
- Managerial Economics Assignment Help
- Econometrics Assignment Help
- Microeconomics Assignment Help
- Business Economics Assignment Help
- Marketing Plan Assignment Help
- Demand Supply Assignment Help
- Comparative Analysis Assignment Help
- Health Economics Assignment Help
- Macroeconomics Assignment Help
- Political Economics Assignment Help
- International Economics Assignments Help
-
Academic Writing Services
-
Essay Writing
- Essay Help
- Essay Writing Help
- Essay Help Online
- Online Custom Essay Help
- Descriptive Essay Help
- Help With MBA Essays
- Essay Writing Service
- Essay Writer For Australia
- Essay Outline Help
- illustration Essay Help
- Response Essay Writing Help
- Professional Essay Writers
- Custom Essay Help
- English Essay Writing Help
- Essay Homework Help
- Literature Essay Help
- Scholarship Essay Help
- Research Essay Help
- History Essay Help
- MBA Essay Help
- Plagiarism Free Essays
- Writing Essay Papers
- Write My Essay Help
- Need Help Writing Essay
- Help Writing Scholarship Essay
- Help Writing a Narrative Essay
- Best Essay Writing Service Canada
-
Dissertation
- Biology Dissertation Help
- Academic Dissertation Help
- Nursing Dissertation Help
- Dissertation Help Online
- MATLAB Dissertation Help
- Doctoral Dissertation Help
- Geography Dissertation Help
- Architecture Dissertation Help
- Statistics Dissertation Help
- Sociology Dissertation Help
- English Dissertation Help
- Law Dissertation Help
- Dissertation Proofreading Services
- Cheap Dissertation Help
- Dissertation Writing Help
- Marketing Dissertation Help
- Programming
-
Case Study
- Write Case Study For Me
- Business Law Case Study Help
- Civil Law Case Study Help
- Marketing Case Study Help
- Nursing Case Study Help
- Case Study Writing Services
- History Case Study help
- Amazon Case Study Help
- Apple Case Study Help
- Case Study Assignment Help
- ZARA Case Study Assignment Help
- IKEA Case Study Assignment Help
- Zappos Case Study Assignment Help
- Tesla Case Study Assignment Help
- Flipkart Case Study Assignment Help
- Contract Law Case Study Assignments Help
- Business Ethics Case Study Assignment Help
- Nike SWOT Analysis Case Study Assignment Help
- Coursework
- Thesis Writing
- CDR
- Research