Order Now
- Home
- About Us
-
Services
-
Assignment Writing
-
Academic Writing Services
- Urgent Assignment Help
- Writing Assignment for University
- College Assignment Help
- SPSS Assignment Help
- HND Assignment Help
- Architecture Assignment Help
- Total Assignment Help
- All Assignment Help
- My Assignment Help
- Student Assignment Help
- Instant Assignment Help
- Cheap Assignment Help
- Global Assignment Help
- Write My Assignment
- Do My Assignment
- Solve My Assignment
- Make My Assignment
- Pay for Assignment Help
-
Management
- Financial Management Assignment Help
- Business Management Assignment Help
- Management Assignment Help
- Project Management Assignment Help
- Supply Chain Management Assignment Help
- Operations Management Assignment Help
- Risk Management Assignment Help
- Strategic Management Assignment Help
- Logistics Management Assignment Help
- Global Business Strategy Assignment Help
- Consumer Behavior Assignment Help
- MBA Assignment Help
- Portfolio Management Assignment Help
- Change Management Assignment Help
- Hospitality Management Assignment Help
- Healthcare Management Assignment Help
- Investment Management Assignment Help
- Market Analysis Assignment Help
- Corporate Strategy Assignment Help
- Conflict Management Assignment Help
- Marketing Management Assignment Help
- Strategic Marketing Assignment Help
- CRM Assignment Help
- Marketing Research Assignment Help
- Human Resource Assignment Help
- Business Assignment Help
- Business Development Assignment Help
- Business Statistics Assignment Help
- Business Ethics Assignment Help
- 4p of Marketing Assignment Help
- Pricing Strategy Assignment Help
- Nursing
-
Finance
- Finance Assignment Help
- Do My Finance Assignment For Me
- Financial Accounting Assignment Help
- Behavioral Finance Assignment Help
- Finance Planning Assignment Help
- Personal Finance Assignment Help
- Financial Services Assignment Help
- Forex Assignment Help
- Financial Statement Analysis Assignment Help
- Capital Budgeting Assignment Help
- Financial Reporting Assignment Help
- International Finance Assignment Help
- Business Finance Assignment Help
- Corporate Finance Assignment Help
-
Accounting
- Accounting Assignment Help
- Managerial Accounting Assignment Help
- Taxation Accounting Assignment Help
- Perdisco Assignment Help
- Solve My Accounting Paper
- Business Accounting Assignment Help
- Cost Accounting Assignment Help
- Taxation Assignment Help
- Activity Based Accounting Assignment Help
- Tax Accounting Assignment Help
- Financial Accounting Theory Assignment Help
-
Computer Science and IT
- Robotics Assignment Help
- Operating System Assignment Help
- Data mining Assignment Help
- Computer Network Assignment Help
- Database Assignment Help
- IT Management Assignment Help
- Network Topology Assignment Help
- Data Structure Assignment Help
- Business Intelligence Assignment Help
- Data Flow Diagram Assignment Help
- UML Diagram Assignment Help
- R Studio Assignment Help
-
Law
- Law Assignment Help
- Business Law Assignment Help
- Contract Law Assignment Help
- Tort Law Assignment Help
- Social Media Law Assignment Help
- Criminal Law Assignment Help
- Employment Law Assignment Help
- Taxation Law Assignment Help
- Commercial Law Assignment Help
- Constitutional Law Assignment Help
- Corporate Governance Law Assignment Help
- Environmental Law Assignment Help
- Criminology Assignment Help
- Company Law Assignment Help
- Human Rights Law Assignment Help
- Evidence Law Assignment Help
- Administrative Law Assignment Help
- Enterprise Law Assignment Help
- Migration Law Assignment Help
- Communication Law Assignment Help
- Law and Ethics Assignment Help
- Consumer Law Assignment Help
- Science
- Biology
- Engineering
-
Humanities
- Humanities Assignment Help
- Sociology Assignment Help
- Philosophy Assignment Help
- English Assignment Help
- Geography Assignment Help
- Agroecology Assignment Help
- Psychology Assignment Help
- Social Science Assignment Help
- Public Relations Assignment Help
- Political Science Assignment Help
- Mass Communication Assignment Help
- History Assignment Help
- Cookery Assignment Help
- Auditing
- Mathematics
-
Economics
- Economics Assignment Help
- Managerial Economics Assignment Help
- Econometrics Assignment Help
- Microeconomics Assignment Help
- Business Economics Assignment Help
- Marketing Plan Assignment Help
- Demand Supply Assignment Help
- Comparative Analysis Assignment Help
- Health Economics Assignment Help
- Macroeconomics Assignment Help
- Political Economics Assignment Help
- International Economics Assignments Help
-
Academic Writing Services
-
Essay Writing
- Essay Help
- Essay Writing Help
- Essay Help Online
- Online Custom Essay Help
- Descriptive Essay Help
- Help With MBA Essays
- Essay Writing Service
- Essay Writer For Australia
- Essay Outline Help
- illustration Essay Help
- Response Essay Writing Help
- Professional Essay Writers
- Custom Essay Help
- English Essay Writing Help
- Essay Homework Help
- Literature Essay Help
- Scholarship Essay Help
- Research Essay Help
- History Essay Help
- MBA Essay Help
- Plagiarism Free Essays
- Writing Essay Papers
- Write My Essay Help
- Need Help Writing Essay
- Help Writing Scholarship Essay
- Help Writing a Narrative Essay
- Best Essay Writing Service Canada
-
Dissertation
- Biology Dissertation Help
- Academic Dissertation Help
- Nursing Dissertation Help
- Dissertation Help Online
- MATLAB Dissertation Help
- Doctoral Dissertation Help
- Geography Dissertation Help
- Architecture Dissertation Help
- Statistics Dissertation Help
- Sociology Dissertation Help
- English Dissertation Help
- Law Dissertation Help
- Dissertation Proofreading Services
- Cheap Dissertation Help
- Dissertation Writing Help
- Marketing Dissertation Help
- Programming
-
Case Study
- Write Case Study For Me
- Business Law Case Study Help
- Civil Law Case Study Help
- Marketing Case Study Help
- Nursing Case Study Help
- Case Study Writing Services
- History Case Study help
- Amazon Case Study Help
- Apple Case Study Help
- Case Study Assignment Help
- ZARA Case Study Assignment Help
- IKEA Case Study Assignment Help
- Zappos Case Study Assignment Help
- Tesla Case Study Assignment Help
- Flipkart Case Study Assignment Help
- Contract Law Case Study Assignments Help
- Business Ethics Case Study Assignment Help
- Nike SWOT Analysis Case Study Assignment Help
- Coursework
- Thesis Writing
- CDR
- Research
-
Assignment Writing
-
Resources
- Referencing Guidelines
-
Universities
-
Australia
- Asia Pacific International College Assignment Help
- Macquarie University Assignment Help
- Rhodes College Assignment Help
- APIC University Assignment Help
- Torrens University Assignment Help
- Kaplan University Assignment Help
- Holmes University Assignment Help
- Griffith University Assignment Help
- VIT University Assignment Help
- CQ University Assignment Help
-
Australia
- Experts
- Free Sample
- Testimonial
MIS609 Data Management and Analytics Case Study Sample
Task Summary
Using the concepts covered in Module 3 and 4, write a 2000 words case study report for a real scenario faced by an organisation of your choice.
Context
This assessment gives you the opportunity to demonstrate your understanding of concepts covered in Module 3 and 4 including Business Intelligence, Big Data, Business Analytics, Data Warehousing, Data Mining, AI, Machine Learning. In doing so, you are required to select an organisation and then analyse and evaluate how the above-mentioned concepts can be used to solve a real-life problem.
Task Instructions
Step 1: Select an organisation that you would like to investigate. When choosing the organisation, make sure that you are able to access data from the organisation easily, or the data is available on the web.
Step 2: Write a case study report outlining how the selected organisation has used the concepts covered in Module 3 and 4 to successfully solve a problem faced by the organisation.
Solution
Section 1
Introduction
Westpac was formed in 1817 and is Australia's oldest bank and corporation. With its current market capitalization, Commonwealth Bank of Australia as well as New Zealand has become one of the world's top banks, including one of the the top ten global publicly traded enterprises (WestPac, 2021). For Assignment Help, Financial services offered by Westpac include retail, business & institutional financing, as well as a high-growth wealth advisory business. In terms of corporate governance and sustainability, Westpac seems to be a worldwide powerhouse. For the past six years, they have been placed top in the Dow Jones Sustainability Index (WestPac, 2021).
Reason for Selection
Considering Westpac Group been around for a long period of time, it was a logical choice. Big customer-related knowledge has been a problem for the organisation in its efforts to use big data analytics to make better business decisions. Since the organisation faced hurdles and gained outcomes, it's best if one learn about how they used big data analytics techniques to overcome those obstacles given their massive database.
Business of Westpac
Westpac Group is a multinational corporation that operates in a number of countries throughout the world. Four customer-focused deals make up the banking group, all of which play a critical role in the company's operations. A wide variety of financial and banking services are offered by Westpac, encompassing wealth management, consumer banking, & institutional banking. Over the course of its global activities, Westpac Group employs about 40,000 people and serves approximately 14 million clients (Li & Wang 2019). Large retail franchise comprising 825 locations and 1,666 ATMs throughout Australia, offering mortgage & credit card and short-long-term deposits.
Section 2
Concepts of Big Data
Unstructured, structured, & semi-organized real-time data are all part of the "big data" idea, which encompasses all types of data. It has to cope with massive, complex data sets which are too large or complex for standard application software to handle. To begin with, it's designed to collect, store, analyse, and then distribute and show data. Professionals and commercial organisations gather useful information from a vast amount of data. Businesses utilise this information to help them make better decisions (Agarwal, 2016). Many major organisations use data to produce real-time improvements in business outcomes and to build a competitive edge in the marketplace among multiple firms. Analyzing data helps to establish frameworks for information management during decision-making. Consequently, company owners will be able to make more informed choices regarding their enterprises.
Business Intelligence
The term "business intelligence" refers to a wide range of technologies that provide quick and simple access to information about an organization's present state based on the available data. BI uses services and tools to translate data into actionable information and help a firm make operational and strategic decisions. Tools for business intelligence access and analyse data sets and show analytical results or breakthroughs in dashboards, charts, reports, graphs, highlights and infographics in order to give users detailed information about the company situation to users (Chandrashekar et al., 2017). The term "business intelligence" refers to a wide range of techniques and concepts that are used to address business-related problems that are beyond the capabilities of human people. An specialist in business intelligence, on the other hand, should be well-versed in the methods, procedures, and technology used to collect and analyse business data. In order to use business intelligence to address problems, those in this position need analytical talents as well (Schoneveld et al., 2021).
Data Warehousing
In the data warehousing idea, there are huge reservoirs of data for combining data from one or many sources into a single location. In a data warehouse, there are specific structures for data storage, processes, and tools that support data quality (Palanivinayagam & Nagarajan, 2020). Deduplication, data extraction, feature extraction, and data integration are only few of the techniques used to assure the integrity of data in the warehouse (Morgan, 2019). Data warehousing has several advantages in terms of technology. An organization's strategic vision and operational efficiency can be improved with the use of these technological advantages.
Data Mining Concept
Patterns may be discovered in enormous databases using the data mining idea. Knowledge of data management, database, and large data is required for data mining. It mostly aids in spotting anomalies in large datasets. It also aids in the understanding of relationships between variables using primary data. Furthermore, data mining aids in the finding of previously unnoticed patterns in large datasets. Data summaries and regression analysis also benefit from this tool (Hussain & Roy, 2016).
Section 3
Data Sources
A wide variety of external data sources were explored to gather the information needed for such an evaluation. The term "secondary data" refers to data gathered by anyone other than the recipient of the information. This material comes from a variety of sources, including the official site of the firm, journal papers, books, and lectures that are available throughout the web. A simpler overview is described below:
Problems Faced
There were a number of issues faced by Westpac Group when it came to collecting and storing data, managing marketing, goods, and services delivery, embezzlement and risk mitigation, absence of appropriate multiple channel experiences, inability to design adequate usage of information strategy, and sharing of data schemes (Cameron, 2014). There wasn't enough assistance from key players for the sector, particularly in terms of finance and board approval for initiatives. In addition to the foregoing, the following critical concerns were discovered:
- Report production or ad hoc queries were typically delayed since data generated by several programmes would have to be manually cleaned, reconciled, and manually coded. Owing to the duplication of work and data, there were also inefficiencies (Cameron, 2014).
- Inconsistent methods for data integration were employed (e.g., push of data into flat files, hand code,. This is also not future-proof because no concepts or approaches were employed to handle emerging Big Data prospects, such as data services as well as service-oriented architecture (SOA).
- There was an error in data handling and data security categories, which resulted in unwarranted risks and possible consequences.
Implementation of the Solution
The Westpac Group was aware that financial services businesses needed to advertise their services and products as a banking sector. When it came to serving consumers and managing customer data across numerous channels, the corporation realised that it had an obligation toward becoming a true, customer-centric organisation. That which was just stated implies that seamless multichannel experiences are available. It was only with the emergence or introduction of big data, notably in the realm of social media, that the bank was able to discover that channel strategies were not restricted to traditional banking channels alone. Before anything else, Westpac set out to establish an information management strategy that would assist them navigate the path of big data (Cameron, 2014). However, achieving such a feat was not without difficulty (WestPac, 2016).
It was determined that the Westpac bank needed better visibility into its data assets. Data warehouse senior System Manager, Mr. Torrance Mayberry recommended Informatica's solution. Torrance Mayberry, a specialist in the field, worked with the bank to help break down organisational barriers and foster a more open and collaborative environment for new ideas. Customer focus was still far off, but Westpac didn't stop exploring the vast possibilities data held, particularly on social media. There was a further increase in the bank's desire to understand its customers in depth. The bank included sentiment analysis within big data analytics as well.
Quddus (2020) said that IBM Banking DW (BDW) was used by Westpac for data warehousing, and that IBM changed the BDW model hybridized version, that was implemented in an Oracle RDBMS, to include the bank's best practises into its DW. As a result, IBM's BDW came to provide a fully standardised and comprehensive representation of the data requirements of said financial services sector. Informatica was the platform of choice for integrating and accessing data. Informatica Metadata Manager was also included in the implementation of Informatica PowerExchange. Informatica platform variant 8.6.1 was used by Westpac until it was updated to edition 9.1 (Quddus, 2020).
An EDW was used as the principal data warehouse architecture, serving as a central hub from which data was fed into a number of smaller data marts that were dependent on it. Analytical solutions for enabling and maximizing economic gain, marketing, including pricing were part of the supply of these data marts. As an example, financial products' price history was saved and managed in the DW, and then sent to the data mart in order to fulfil the analysis requirements for pricing rationalization. Informatica's platform gathered data from the bank's PRM system, that allowed this same bank to quickly refresh its knowledge of fraud and reduce individual risk. Data-driven decision-making at Westpac developed as a result of increased trust in the information provided by the DW, as well as the creation of new business links.
Section 4
Problems Faced in Implementation
Data warehousing (DW) was the first step in Westpac's road to Big Data. Similarly to other large corporations with data-intensive tasks, the Westpac Group has a large number of unconnected apps that were not meant to share information.
- There was a lack of data strategy. The lack of a single version of Westpac's products and clients because critical data was gathered and stored in silos, and dissimilar information utilisation and definitions were the norm; and the inability to obtain a single version of Westpac's products and clients because critical data was gathered and stored in silos, and dissimilar information utilisation and definitions were the norm (Cameron, 2014).
- Due to the laborious scrubbing, validation, and hand-coding of data from several systems, the response time for ad hoc or reporting production requests was sometimes delayed. In addition, there was a lack of efficiency due to the duplication of data and labour
- To integrate data, many methods were used, including pushing information into flat files and manually coding.
- Soa (service-oriented architecture) as well as data services didn't exist at the time; and there were no methodologies or ideas that might be used to handle new big data opportunities; Information security and data handling were classified wrongly, resulting in potentially harmful complications and hazards.
Benefits Realization to WestPac
According to Quddus (2020), Westpac has reaped the benefits of the big data revolution in a variety of ways. According to Westpac's data warehouse, a large number of its business units (LOBs) are now able to get information as well as reports from it (DW). Westpac said that the bank's core business stakeholders started to realise the strategic importance of the bank's data assets therefore began to embrace Westpac DW acceleration. Financing, retail and commercial banking departments in both New Zealand and Australia as well as an insight team for risk analytics as well as customer service were all part of it. It was possible for Westpac to invest in the development of a comprehensive data strategy to guide business decisions by delivering relevant, accurate, comprehensive, on-time managed information and data through the implementation of this plan. Impacted quantifiable goals and results for securing top-level management help as a result of change. In Westpac's view, the project's goals and outcomes were viewed as data-driven. The chance of obtaining funds and board approval for such ventures grew in respect of profit and productivity.
Conclusion
Big data analytics have just placed the company in a functioning stage thanks to the potential future gains or expansions that may be derived from the examination of enormous amounts of data. The Westpac Group anticipates the big data analysis journey to help the banking industry obtain insights according to what clients are saying, what they are seeking for, or what kinds of issues they are facing. The bank will be able to create, advertise, and sell more effective services, programmes, and products as a result of this.
Recommendations
The following recommendations can be sought for
- These early victories are vital.
DW team at Westpac was able to leverage this accomplishment to involve key stakeholders as well as the (LOBS) or line of businesses, thereby increasing awareness of the problem for the company's data strategy by leveraging this internal success.
- To obtain the support of the company's senior management, provide a set of quantifiable goals.
Westpac correctly recognises that quantifying the aims and outcomes of data-centric projects in order to improve productivity and profit enhances the likelihood that certain projects will be approved by the board and funded.
- Enhance IT and business cooperation.
"Lost in translation" problems can be avoided if IT and business people work together effectively.
References
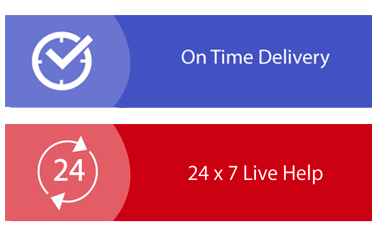
Download Samples PDF
Related Sample
- MITS5002 Software Engineering Methodology Assignment
- ICT500 Emerging Technologies Report 3
- ENT201 Sales and Negotiation Strategies Assignment
- MIS609 Data Management and Analytics Assignment
- BUECO5903 Assignment
- Pure Gym 202202 Marketing Mid term Coursework
- BSBSUS511 Develop Workplace Policy and Procedure Assignment
- Audit Quality Essay on Australian Parliamentary Committee Assignment
- ITECH3001 Evaluating a System Assignment
- 2105AFE Introduction To Business Law Assignment
- PUBH6206 Health Promotion and Community Health Assignment
- Finance Coursework Assignment
- EBP107 Evidence Based Practice Assignment
- MIS603 Microservices Architecture Report 2
- BSBITU404 Produce Complex Desktop Published Document Assignment
- MGT607 Innovation Creativity and Entrepreneurship Report
- MGT502 Business Communication
- Gonocytes Coursework Assignment
- MGT602 Business Decision Analytics Assignment 2
- Advanced Network Design Assignment
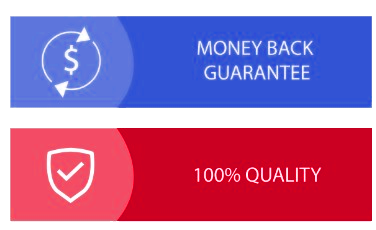
Assignment Services
-
Assignment Writing
-
Academic Writing Services
- Urgent Assignment Help
- Writing Assignment for University
- College Assignment Help
- SPSS Assignment Help
- HND Assignment Help
- Architecture Assignment Help
- Total Assignment Help
- All Assignment Help
- My Assignment Help
- Student Assignment Help
- Instant Assignment Help
- Cheap Assignment Help
- Global Assignment Help
- Write My Assignment
- Do My Assignment
- Solve My Assignment
- Make My Assignment
- Pay for Assignment Help
-
Management
- Financial Management Assignment Help
- Business Management Assignment Help
- Management Assignment Help
- Project Management Assignment Help
- Supply Chain Management Assignment Help
- Operations Management Assignment Help
- Risk Management Assignment Help
- Strategic Management Assignment Help
- Logistics Management Assignment Help
- Global Business Strategy Assignment Help
- Consumer Behavior Assignment Help
- MBA Assignment Help
- Portfolio Management Assignment Help
- Change Management Assignment Help
- Hospitality Management Assignment Help
- Healthcare Management Assignment Help
- Investment Management Assignment Help
- Market Analysis Assignment Help
- Corporate Strategy Assignment Help
- Conflict Management Assignment Help
- Marketing Management Assignment Help
- Strategic Marketing Assignment Help
- CRM Assignment Help
- Marketing Research Assignment Help
- Human Resource Assignment Help
- Business Assignment Help
- Business Development Assignment Help
- Business Statistics Assignment Help
- Business Ethics Assignment Help
- 4p of Marketing Assignment Help
- Pricing Strategy Assignment Help
- Nursing
-
Finance
- Finance Assignment Help
- Do My Finance Assignment For Me
- Financial Accounting Assignment Help
- Behavioral Finance Assignment Help
- Finance Planning Assignment Help
- Personal Finance Assignment Help
- Financial Services Assignment Help
- Forex Assignment Help
- Financial Statement Analysis Assignment Help
- Capital Budgeting Assignment Help
- Financial Reporting Assignment Help
- International Finance Assignment Help
- Business Finance Assignment Help
- Corporate Finance Assignment Help
-
Accounting
- Accounting Assignment Help
- Managerial Accounting Assignment Help
- Taxation Accounting Assignment Help
- Perdisco Assignment Help
- Solve My Accounting Paper
- Business Accounting Assignment Help
- Cost Accounting Assignment Help
- Taxation Assignment Help
- Activity Based Accounting Assignment Help
- Tax Accounting Assignment Help
- Financial Accounting Theory Assignment Help
-
Computer Science and IT
- Robotics Assignment Help
- Operating System Assignment Help
- Data mining Assignment Help
- Computer Network Assignment Help
- Database Assignment Help
- IT Management Assignment Help
- Network Topology Assignment Help
- Data Structure Assignment Help
- Business Intelligence Assignment Help
- Data Flow Diagram Assignment Help
- UML Diagram Assignment Help
- R Studio Assignment Help
-
Law
- Law Assignment Help
- Business Law Assignment Help
- Contract Law Assignment Help
- Tort Law Assignment Help
- Social Media Law Assignment Help
- Criminal Law Assignment Help
- Employment Law Assignment Help
- Taxation Law Assignment Help
- Commercial Law Assignment Help
- Constitutional Law Assignment Help
- Corporate Governance Law Assignment Help
- Environmental Law Assignment Help
- Criminology Assignment Help
- Company Law Assignment Help
- Human Rights Law Assignment Help
- Evidence Law Assignment Help
- Administrative Law Assignment Help
- Enterprise Law Assignment Help
- Migration Law Assignment Help
- Communication Law Assignment Help
- Law and Ethics Assignment Help
- Consumer Law Assignment Help
- Science
- Biology
- Engineering
-
Humanities
- Humanities Assignment Help
- Sociology Assignment Help
- Philosophy Assignment Help
- English Assignment Help
- Geography Assignment Help
- Agroecology Assignment Help
- Psychology Assignment Help
- Social Science Assignment Help
- Public Relations Assignment Help
- Political Science Assignment Help
- Mass Communication Assignment Help
- History Assignment Help
- Cookery Assignment Help
- Auditing
- Mathematics
-
Economics
- Economics Assignment Help
- Managerial Economics Assignment Help
- Econometrics Assignment Help
- Microeconomics Assignment Help
- Business Economics Assignment Help
- Marketing Plan Assignment Help
- Demand Supply Assignment Help
- Comparative Analysis Assignment Help
- Health Economics Assignment Help
- Macroeconomics Assignment Help
- Political Economics Assignment Help
- International Economics Assignments Help
-
Academic Writing Services
-
Essay Writing
- Essay Help
- Essay Writing Help
- Essay Help Online
- Online Custom Essay Help
- Descriptive Essay Help
- Help With MBA Essays
- Essay Writing Service
- Essay Writer For Australia
- Essay Outline Help
- illustration Essay Help
- Response Essay Writing Help
- Professional Essay Writers
- Custom Essay Help
- English Essay Writing Help
- Essay Homework Help
- Literature Essay Help
- Scholarship Essay Help
- Research Essay Help
- History Essay Help
- MBA Essay Help
- Plagiarism Free Essays
- Writing Essay Papers
- Write My Essay Help
- Need Help Writing Essay
- Help Writing Scholarship Essay
- Help Writing a Narrative Essay
- Best Essay Writing Service Canada
-
Dissertation
- Biology Dissertation Help
- Academic Dissertation Help
- Nursing Dissertation Help
- Dissertation Help Online
- MATLAB Dissertation Help
- Doctoral Dissertation Help
- Geography Dissertation Help
- Architecture Dissertation Help
- Statistics Dissertation Help
- Sociology Dissertation Help
- English Dissertation Help
- Law Dissertation Help
- Dissertation Proofreading Services
- Cheap Dissertation Help
- Dissertation Writing Help
- Marketing Dissertation Help
- Programming
-
Case Study
- Write Case Study For Me
- Business Law Case Study Help
- Civil Law Case Study Help
- Marketing Case Study Help
- Nursing Case Study Help
- Case Study Writing Services
- History Case Study help
- Amazon Case Study Help
- Apple Case Study Help
- Case Study Assignment Help
- ZARA Case Study Assignment Help
- IKEA Case Study Assignment Help
- Zappos Case Study Assignment Help
- Tesla Case Study Assignment Help
- Flipkart Case Study Assignment Help
- Contract Law Case Study Assignments Help
- Business Ethics Case Study Assignment Help
- Nike SWOT Analysis Case Study Assignment Help
- Coursework
- Thesis Writing
- CDR
- Research