Order Now
- Home
- About Us
-
Services
-
Assignment Writing
-
Academic Writing Services
- HND Assignment Help
- SPSS Assignment Help
- College Assignment Help
- Writing Assignment for University
- Urgent Assignment Help
- Architecture Assignment Help
- Total Assignment Help
- All Assignment Help
- My Assignment Help
- Student Assignment Help
- Instant Assignment Help
- Cheap Assignment Help
- Global Assignment Help
- Write My Assignment
- Do My Assignment
- Solve My Assignment
- Make My Assignment
- Pay for Assignment Help
-
Management
- Management Assignment Help
- Business Management Assignment Help
- Financial Management Assignment Help
- Project Management Assignment Help
- Supply Chain Management Assignment Help
- Operations Management Assignment Help
- Risk Management Assignment Help
- Strategic Management Assignment Help
- Logistics Management Assignment Help
- Global Business Strategy Assignment Help
- Consumer Behavior Assignment Help
- MBA Assignment Help
- Portfolio Management Assignment Help
- Change Management Assignment Help
- Hospitality Management Assignment Help
- Healthcare Management Assignment Help
- Investment Management Assignment Help
- Market Analysis Assignment Help
- Corporate Strategy Assignment Help
- Conflict Management Assignment Help
- Marketing Management Assignment Help
- Strategic Marketing Assignment Help
- CRM Assignment Help
- Marketing Research Assignment Help
- Human Resource Assignment Help
- Business Assignment Help
- Business Development Assignment Help
- Business Statistics Assignment Help
- Business Ethics Assignment Help
- 4p of Marketing Assignment Help
- Pricing Strategy Assignment Help
- Nursing
-
Finance
- Finance Assignment Help
- Do My Finance Assignment For Me
- Financial Accounting Assignment Help
- Behavioral Finance Assignment Help
- Finance Planning Assignment Help
- Personal Finance Assignment Help
- Financial Services Assignment Help
- Forex Assignment Help
- Financial Statement Analysis Assignment Help
- Capital Budgeting Assignment Help
- Financial Reporting Assignment Help
- International Finance Assignment Help
- Business Finance Assignment Help
- Corporate Finance Assignment Help
-
Accounting
- Accounting Assignment Help
- Managerial Accounting Assignment Help
- Taxation Accounting Assignment Help
- Perdisco Assignment Help
- Solve My Accounting Paper
- Business Accounting Assignment Help
- Cost Accounting Assignment Help
- Taxation Assignment Help
- Activity Based Accounting Assignment Help
- Tax Accounting Assignment Help
- Financial Accounting Theory Assignment Help
-
Computer Science and IT
- Operating System Assignment Help
- Data mining Assignment Help
- Robotics Assignment Help
- Computer Network Assignment Help
- Database Assignment Help
- IT Management Assignment Help
- Network Topology Assignment Help
- Data Structure Assignment Help
- Business Intelligence Assignment Help
- Data Flow Diagram Assignment Help
- UML Diagram Assignment Help
- R Studio Assignment Help
-
Law
- Law Assignment Help
- Business Law Assignment Help
- Contract Law Assignment Help
- Tort Law Assignment Help
- Social Media Law Assignment Help
- Criminal Law Assignment Help
- Employment Law Assignment Help
- Taxation Law Assignment Help
- Commercial Law Assignment Help
- Constitutional Law Assignment Help
- Corporate Governance Law Assignment Help
- Environmental Law Assignment Help
- Criminology Assignment Help
- Company Law Assignment Help
- Human Rights Law Assignment Help
- Evidence Law Assignment Help
- Administrative Law Assignment Help
- Enterprise Law Assignment Help
- Migration Law Assignment Help
- Communication Law Assignment Help
- Law and Ethics Assignment Help
- Consumer Law Assignment Help
- Science
- Biology
- Engineering
-
Humanities
- Humanities Assignment Help
- Sociology Assignment Help
- Philosophy Assignment Help
- English Assignment Help
- Geography Assignment Help
- Agroecology Assignment Help
- Psychology Assignment Help
- Social Science Assignment Help
- Public Relations Assignment Help
- Political Science Assignment Help
- Mass Communication Assignment Help
- History Assignment Help
- Cookery Assignment Help
- Auditing
- Mathematics
-
Economics
- Economics Assignment Help
- Managerial Economics Assignment Help
- Econometrics Assignment Help
- Microeconomics Assignment Help
- Business Economics Assignment Help
- Marketing Plan Assignment Help
- Demand Supply Assignment Help
- Comparative Analysis Assignment Help
- Health Economics Assignment Help
- Macroeconomics Assignment Help
- Political Economics Assignment Help
- International Economics Assignments Help
-
Academic Writing Services
-
Essay Writing
- Essay Help
- Essay Writing Help
- Essay Help Online
- Online Custom Essay Help
- Descriptive Essay Help
- Help With MBA Essays
- Essay Writing Service
- Essay Writer For Australia
- Essay Outline Help
- illustration Essay Help
- Response Essay Writing Help
- Professional Essay Writers
- Custom Essay Help
- English Essay Writing Help
- Essay Homework Help
- Literature Essay Help
- Scholarship Essay Help
- Research Essay Help
- History Essay Help
- MBA Essay Help
- Plagiarism Free Essays
- Writing Essay Papers
- Write My Essay Help
- Need Help Writing Essay
- Help Writing Scholarship Essay
- Help Writing a Narrative Essay
- Best Essay Writing Service Canada
-
Dissertation
- Biology Dissertation Help
- Academic Dissertation Help
- Nursing Dissertation Help
- Dissertation Help Online
- MATLAB Dissertation Help
- Doctoral Dissertation Help
- Geography Dissertation Help
- Architecture Dissertation Help
- Statistics Dissertation Help
- Sociology Dissertation Help
- English Dissertation Help
- Law Dissertation Help
- Dissertation Proofreading Services
- Cheap Dissertation Help
- Dissertation Writing Help
- Marketing Dissertation Help
- Programming
-
Case Study
- Write Case Study For Me
- Business Law Case Study Help
- Civil Law Case Study Help
- Marketing Case Study Help
- Nursing Case Study Help
- Case Study Writing Services
- History Case Study help
- Amazon Case Study Help
- Apple Case Study Help
- Case Study Assignment Help
- ZARA Case Study Assignment Help
- IKEA Case Study Assignment Help
- Zappos Case Study Assignment Help
- Tesla Case Study Assignment Help
- Flipkart Case Study Assignment Help
- Contract Law Case Study Assignments Help
- Business Ethics Case Study Assignment Help
- Nike SWOT Analysis Case Study Assignment Help
- Coursework
- Thesis Writing
- CDR
- Research
-
Assignment Writing
-
Resources
- Referencing Guidelines
-
Universities
-
Australia
- Asia Pacific International College Assignment Help
- Macquarie University Assignment Help
- Rhodes College Assignment Help
- APIC University Assignment Help
- Torrens University Assignment Help
- Kaplan University Assignment Help
- Holmes University Assignment Help
- Griffith University Assignment Help
- VIT University Assignment Help
- CQ University Assignment Help
-
Australia
- Experts
- Free Sample
- Testimonial
STAT6200 Statistics for Public Health Assignment Help
Assignment Brief
Individual/Group - Individual
Length - 1,200 words (+/- 10%)
Learning Outcomes
This assessment addresses the following learning outcomes:
a) Critically apply the theories on key concepts in descriptive and inferential statistics
b) Analyze survey design and sampling methods to collect valid and reliable data and appraise methodologies
c) Assess the data and determine the appropriate parametric and non-parametric statistical tests, and how to control for confounding variables
d) Evaluate types of inferential statistics and interpret the results of these analyses using theoretical examples or as presented in published literature
e) Apply key concepts of statistics, including: sampling, hypothesis testing, distribution of data, validity and reliability, statistical significance and effect size
Submission Due Sunday following the end of Module 8 at 11:55pm
Weighting - 40%
Total Marks - 100 marks
Instructions:
This assessment requires you to read excerpts from four articles and answer a series of questions in no more than 1,200 words (+/- 10%).
Most public health and wider health science journals report some form of statistics. The ability to understand and extract meaning from journal articles, and the ability to critically evaluate the statistics reported in research papers are fundamental skills in public health. This type of assessment demonstrates how students can apply the skills that they learn in this course to real-world scenarios wherein they might need to interpret/review articles for public health use.
After reading published research articles, you will be asked to interpret, describe and report the following types of statistics for assignment help:
o State the null and alternative hypothesis
o Detail the demographic characteristics of the people in a sample
o Report summary descriptive and inferential statistics reported in the paper
o Describe what inferential statistics were used for the analysis of data in a study and why
o Interpret the odds ratios or hazard ratios for reported outcomes
o Evaluate the impact design limitations described by the researchers have on study or the extent to which results can be generalized to the population
Paper Excerpts for Interpretation
Paper 1:
1. What was the purpose of the research?
2. What kind of data was used, and what statistical analysis was performed on the data?
3. Refer to Table 2. Describe the correlation between overweight, obesity, BMI and HDI for both men and women.
4. What inferential statistics were used for analysis of the data summarized in Table 2, and why?
5. What was the conclusion of the study?
Paper 2:
1. Describe the research design of the study.
2. What demographic characteristics were considered for the people in the sample? Explain by referring to the descriptive statistics reported in the paper.
3. Which reported statistic was common to Figures 2, 4 and 5 in this paper? Please describe the outcome variable and statistic.
4. Refer to Figure 2. What did the researchers find for the number of disease-free years from age 40 when they categorized the participants by BMI?
5. What type of descriptive statistic is illustrated in Figure 3? List the variables included.
Paper 3:
1. Describe the aim of the study. Based on the study design, can the aim be restated in terms of null and alternative hypotheses?
2. What type of statistical analysis was used to independently examine the effect of diabetes status and type on in-hospital death with COVID-19? Why?
3. Interpret and report the adjusted odds ratios for in-hospital COVID-19-related death associated with diabetes status.
4. How generalizable are the findings described by the researchers to the population, and why?
5. What was the main finding of the study?
Paper 4:
1. Describe the aims of the study. Based on the study design, can the aim be restated in terms of null and alternative hypotheses? If so, state the null and alternative hypothesis.
2. Which four primary outcomes were examined by the study? Refer to Figure 2 - interpret and report the statistical results of these four primary outcomes.
3. Examine Figure 3, what was the outcome for Diabetes? Please report the statistics.
4. What was the most likely explanation for the effect of the intervention described in the discussion?
5. What were the limitations of the study reported in the discussion?
Solution
Paper 1:
Previous researches have demonstrated that obesity is one of the known health threats that lead to serious non-communicable diseases like diabetes, cardiovascular disease, blood pressure and even cancer. Ataey et al. (2020), arguesin their study that Human Development Index (HDI) has significant impact on the prevalence of obesity. The study also assesses the degree to which HDI influence the prevalence of overweight and obesity.
In the analysis, Ataey et al. (2020) used secondary quantitative data and using the SPSS, descriptive and inferential statistical analysis has been used. To determine the association between prevalence of overweight and obesity with HDI based on gender, data for Eastern Mediterranean Region has been collected. UN resource for HDI data and WHO resource were used for gathering information regarding overweight, obesity and other non-communicable diseases.
The study has presented its finding in table 2 where correlation between HDI with overweight, obesity and BMI is presented. As per the correlation, for male HDI has significant impact on overweight, obesity and BMI as their p value is lower than 0.05. Correlation also demonstrates the HDI influence the overweight, obesity and BMI highly as correlation of .721, .714 and .549 shows, HDI can lead to change in overweight by 72.1%, 71.4% and 54.9% respectively (Ataey et al. 2020). When it comes to female, then the correlation is valid for overweight and obesity as their p value is lower than 0.05. Correlation of .615 and .617 demonstrates, HDI can change overweight by 61.5% and obesity by 61.7% respectively (Ataey et al. 2020). Hence, for as the inferential analysis, here correlation analysis has been used to summarise the outcome in table 2.
To conclude, the study stated that there is good level of significant association between HDI and obesity and overweight (Ataey et al. 2020). The study at last asks policymakers to consider HDI factors while making general health policy for controlling non-communicable diseases.
Paper 2:
Previous studies have demonstrated that obesity is one of the major factors that lead to risks of several chronic diseases. However, it is not yet discovered, to which extent obesity is connected with the loss of diseases free years in different socioeconomic groups. Thus, the study authored by Nyberg et al. (2018) has performed a comparative analysis to determine the number of free years from any non-communicable disease for people overweight and obese with people who are normal weight. To analyse the research aim, present study has used quantitative approach of data analysis. Using the deductive approach of study, here analysis has been done of the Body Mass Index (BMI) with risk free years based on gender. To perform the study, researcher here considered variables like BMI, gender and age.
As per the statistical analysis, it can be seen that mean age of the male is 44.6 years and it is 43.4 years for female. Out of total respondents, 60.8% were female respondents and 39.2% were male respondents (Nyberg et al. 2018). Average BMI is 25.7 kg/m2 for males, and BMI is 21.468 for normal weight and 14.93 kg/m2 for overweight males. On the other hand, for females, mean BMI is 24.5 kg/m2 with 44.760 for normal weight and 5.670 for overweight (Nyberg et al. 2018).
Coming to the finding of the analysis, it has been observed that figure 2, 4 and 5 demonstrates mean age of disease-free years. Also, all three figures demonstrate gender wise significance of the disease-free years. As per the figure 2, it can be seen that when participants were arranged based by BMI, then average men age free year is 69.3 years for normal weight and 65.3 years for obese level I (Nyberg et al. 2018). For women, risk free years is 69.4 years and it is 66.7 years for obese level I (Nyberg et al. 2018). Using the descriptive statistics, prevalence level of obesity, has been presented based on socioeconomic status and gender. Hence, here graphical presentation has been used as descriptive statistics. Variable that was included in the study were obesity level, smoking, physical inactivity, gender and socio-economic status.
Paper 3:
Various study has demonstrated that there is long standing debate that whether diabetes has association with morality related to covid19. To understand the phenomenon, analysis has been made to check how the relative risks for type 1 and type 2 diabetes impact the covid19 related mortality during march 1 2020 to May 11 2020 by (Barron et al. 2020). Underpinning the study design, the study aim can be restated as well like: how the mortality rate of covid19 is associated with the diabetes type 1 and type 2. To analyse the restated research aim, hypothesis can be developed as follows:
Null hypothesis (H0): Mortality rate of covid19 does not have association with type 1 and type 2 diabetes
Alternative hypothesis (H1): Mortality rate of covid19 have positive association with type 1 and type 2 diabetes
Using the actual research design, for analysing the effect of diabetes and the type of in-hospital death during covid19, odd ratio has been used and descriptive statistics were used to determine generic characteristics of the data. In figure 2, the study has presented adjusted odd ratios for demonstrating in-hospital covid19 related death for diabetes. As per the same, it can be seen that odd ratio is high for female and people who are ages more than 80 years and who have diabetes have odd ratio of 9.2 compared to control group. It has been further found that people who have diabetes type 1 are more prone to covid19 mortality and for type 2 diabetes people mortality rate is low (Barron et al. 2020). The finding of the study is valid for the England population who are having type 1 and type 2 diabetes. Thus, the study finding cannot be generalised for other part of the world where the factors like ethnicity, diabetes level, age benchmark changes. To conclude, the study found that there is independent association between the covid19 mortality and in hospital death for type 1 and type 2 diabetes.
Paper 4:
Previous studies have demonstrated that lifestyle intervention can slow down the type 2 diabetes glucose tolerance level; however, it is uncertain that whether it result in fewer complication or enhanced longevity under uncertainty. Thus, Gong et al. (2019) authored their study to determine how long-term effect of lifestyle intervention with impaired glucose tolerance level have implication on diabetes and mortality. Based on the study design, it can be restated as null and alternative hypothesis.
Null hypothesis: there is no significant influence of lifestyle intervention in people with impaired glucose tolerance level on diabetes and mortality
Alternative hypothesis: there is positive significant influence of lifestyle intervention in people with impaired glucose tolerance level on diabetes and mortality
To analyse the association, four major variables were considered, as presented in figure 2, which diabetes, cardiovascular disease (CVD) event, CVD deaths and Compositive microvascular disease. Figure 2 demonstrated the difference in diabetes and mortality effect on control and intervention group. The finding demonstrated that intervention group has media delays in diabetes by 3.96 years (Gong et al. 2019). CVD event and CVD deaths represented a 1.44-year growth in life expectancy. Figure 3 finding demonstrates that intervention group has better hazard ratio during follow up time and significance level was 0.001. For the CVD event, CVD death, Composite microvascular disease also, intervention group demonstrated better outcome. Though the finding was good, it was limited in terms of size of sample. Apart from this, there was irregularities in participation examination and the finding was limited for diabetes type 2 patients only.
Reference:
Ataey, A., Jafarvand, E., Adham, D., & Moradi-Asl, E. (2020). The relationship between obesity, overweight, and the human development index in world health organization eastern Mediterranean region countries. Journal of Preventive Medicine and Public Health, 53(2), 98. https://www.ncbi.nlm.nih.gov/pmc/articles/PMC7142010/
Barron, E., Bakhai, C., Kar, P., Weaver, A., Bradley, D., Ismail, H., ... & Valabhji, J. (2020). Associations of type 1 and type 2 diabetes with COVID-19-related mortality in England: a whole-population study. The lancet Diabetes & endocrinology, 8(10), 813-822. https://www.ncbi.nlm.nih.gov/pmc/articles/pmc7426088/
Gong, Q., Zhang, P., Wang, J., Ma, J., An, Y., Chen, Y., ... & Roglic, G. (2019). Morbidity and mortality after lifestyle intervention for people with impaired glucose tolerance: 30-year results of the Da Qing Diabetes Prevention Outcome Study. The lancet Diabetes & endocrinology, 7(6), 452-461. https://www.ncbi.nlm.nih.gov/pmc/articles/pmc8172050/
Nyberg, S. T., Batty, G. D., Pentti, J., Virtanen, M., Alfredsson, L., Fransson, E. I., ... & Kivimäki, M. (2018). Obesity and loss of disease-free years owing to major non-communicable diseases: a multicohort study. The lancet Public health, 3(10), e490-e497. https://www.sciencedirect.com/science/article/pii/S2468266718301397
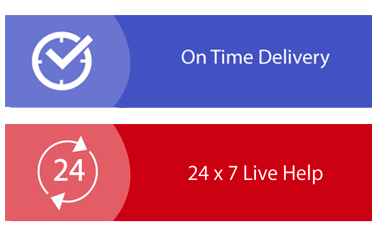
Download Samples PDF
Related Sample
- ICC104 Introduction to Cloud Computing Case Study 2
- DATA4700 Digital Marketing and Competitive Advantage Report 3
- COIT20262 Advanced Network Security Assignment
- Novel Protein Acherons Distribution Using Fluorescence Microscopy Assignment
- PROJ6004 Contracts and Procurement Case Study
- Managing Information Systems Technology Projects
- MGN428 Developing Entrepreneurial Mindsets Assignment
- HDS310 Human Rights and Advocacy Assignment
- MIS500 Foundations of Information Systems Report 3
- MBA6304 Hospitality Management Report 3
- MGT604 Strategic Management Report
- BSBINS603 Initiate and Lead Applied Research Assignment
- Big Data and Analytics Assignment
- EDUC1001 Language and Learning in Your Discipline Assignment
- LAW6001 Taxation Law Case Study 2
- PROG2008 Computational Thinking Assignment 3
- PRJ6001 Applied Project Report 1
- ACC2CRE Financial Accounting and Reporting Assignment
- PRJ5106 Research Methodology and Data Analysis Report 1
- ICT80008 Professional Issues in IT Assignment
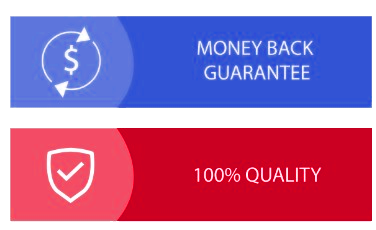
Assignment Services
-
Assignment Writing
-
Academic Writing Services
- HND Assignment Help
- SPSS Assignment Help
- College Assignment Help
- Writing Assignment for University
- Urgent Assignment Help
- Architecture Assignment Help
- Total Assignment Help
- All Assignment Help
- My Assignment Help
- Student Assignment Help
- Instant Assignment Help
- Cheap Assignment Help
- Global Assignment Help
- Write My Assignment
- Do My Assignment
- Solve My Assignment
- Make My Assignment
- Pay for Assignment Help
-
Management
- Management Assignment Help
- Business Management Assignment Help
- Financial Management Assignment Help
- Project Management Assignment Help
- Supply Chain Management Assignment Help
- Operations Management Assignment Help
- Risk Management Assignment Help
- Strategic Management Assignment Help
- Logistics Management Assignment Help
- Global Business Strategy Assignment Help
- Consumer Behavior Assignment Help
- MBA Assignment Help
- Portfolio Management Assignment Help
- Change Management Assignment Help
- Hospitality Management Assignment Help
- Healthcare Management Assignment Help
- Investment Management Assignment Help
- Market Analysis Assignment Help
- Corporate Strategy Assignment Help
- Conflict Management Assignment Help
- Marketing Management Assignment Help
- Strategic Marketing Assignment Help
- CRM Assignment Help
- Marketing Research Assignment Help
- Human Resource Assignment Help
- Business Assignment Help
- Business Development Assignment Help
- Business Statistics Assignment Help
- Business Ethics Assignment Help
- 4p of Marketing Assignment Help
- Pricing Strategy Assignment Help
- Nursing
-
Finance
- Finance Assignment Help
- Do My Finance Assignment For Me
- Financial Accounting Assignment Help
- Behavioral Finance Assignment Help
- Finance Planning Assignment Help
- Personal Finance Assignment Help
- Financial Services Assignment Help
- Forex Assignment Help
- Financial Statement Analysis Assignment Help
- Capital Budgeting Assignment Help
- Financial Reporting Assignment Help
- International Finance Assignment Help
- Business Finance Assignment Help
- Corporate Finance Assignment Help
-
Accounting
- Accounting Assignment Help
- Managerial Accounting Assignment Help
- Taxation Accounting Assignment Help
- Perdisco Assignment Help
- Solve My Accounting Paper
- Business Accounting Assignment Help
- Cost Accounting Assignment Help
- Taxation Assignment Help
- Activity Based Accounting Assignment Help
- Tax Accounting Assignment Help
- Financial Accounting Theory Assignment Help
-
Computer Science and IT
- Operating System Assignment Help
- Data mining Assignment Help
- Robotics Assignment Help
- Computer Network Assignment Help
- Database Assignment Help
- IT Management Assignment Help
- Network Topology Assignment Help
- Data Structure Assignment Help
- Business Intelligence Assignment Help
- Data Flow Diagram Assignment Help
- UML Diagram Assignment Help
- R Studio Assignment Help
-
Law
- Law Assignment Help
- Business Law Assignment Help
- Contract Law Assignment Help
- Tort Law Assignment Help
- Social Media Law Assignment Help
- Criminal Law Assignment Help
- Employment Law Assignment Help
- Taxation Law Assignment Help
- Commercial Law Assignment Help
- Constitutional Law Assignment Help
- Corporate Governance Law Assignment Help
- Environmental Law Assignment Help
- Criminology Assignment Help
- Company Law Assignment Help
- Human Rights Law Assignment Help
- Evidence Law Assignment Help
- Administrative Law Assignment Help
- Enterprise Law Assignment Help
- Migration Law Assignment Help
- Communication Law Assignment Help
- Law and Ethics Assignment Help
- Consumer Law Assignment Help
- Science
- Biology
- Engineering
-
Humanities
- Humanities Assignment Help
- Sociology Assignment Help
- Philosophy Assignment Help
- English Assignment Help
- Geography Assignment Help
- Agroecology Assignment Help
- Psychology Assignment Help
- Social Science Assignment Help
- Public Relations Assignment Help
- Political Science Assignment Help
- Mass Communication Assignment Help
- History Assignment Help
- Cookery Assignment Help
- Auditing
- Mathematics
-
Economics
- Economics Assignment Help
- Managerial Economics Assignment Help
- Econometrics Assignment Help
- Microeconomics Assignment Help
- Business Economics Assignment Help
- Marketing Plan Assignment Help
- Demand Supply Assignment Help
- Comparative Analysis Assignment Help
- Health Economics Assignment Help
- Macroeconomics Assignment Help
- Political Economics Assignment Help
- International Economics Assignments Help
-
Academic Writing Services
-
Essay Writing
- Essay Help
- Essay Writing Help
- Essay Help Online
- Online Custom Essay Help
- Descriptive Essay Help
- Help With MBA Essays
- Essay Writing Service
- Essay Writer For Australia
- Essay Outline Help
- illustration Essay Help
- Response Essay Writing Help
- Professional Essay Writers
- Custom Essay Help
- English Essay Writing Help
- Essay Homework Help
- Literature Essay Help
- Scholarship Essay Help
- Research Essay Help
- History Essay Help
- MBA Essay Help
- Plagiarism Free Essays
- Writing Essay Papers
- Write My Essay Help
- Need Help Writing Essay
- Help Writing Scholarship Essay
- Help Writing a Narrative Essay
- Best Essay Writing Service Canada
-
Dissertation
- Biology Dissertation Help
- Academic Dissertation Help
- Nursing Dissertation Help
- Dissertation Help Online
- MATLAB Dissertation Help
- Doctoral Dissertation Help
- Geography Dissertation Help
- Architecture Dissertation Help
- Statistics Dissertation Help
- Sociology Dissertation Help
- English Dissertation Help
- Law Dissertation Help
- Dissertation Proofreading Services
- Cheap Dissertation Help
- Dissertation Writing Help
- Marketing Dissertation Help
- Programming
-
Case Study
- Write Case Study For Me
- Business Law Case Study Help
- Civil Law Case Study Help
- Marketing Case Study Help
- Nursing Case Study Help
- Case Study Writing Services
- History Case Study help
- Amazon Case Study Help
- Apple Case Study Help
- Case Study Assignment Help
- ZARA Case Study Assignment Help
- IKEA Case Study Assignment Help
- Zappos Case Study Assignment Help
- Tesla Case Study Assignment Help
- Flipkart Case Study Assignment Help
- Contract Law Case Study Assignments Help
- Business Ethics Case Study Assignment Help
- Nike SWOT Analysis Case Study Assignment Help
- Coursework
- Thesis Writing
- CDR
- Research