Order Now
- Home
- About Us
-
Services
-
Assignment Writing
-
Academic Writing Services
- Urgent Assignment Help
- Writing Assignment for University
- College Assignment Help
- SPSS Assignment Help
- HND Assignment Help
- Architecture Assignment Help
- Total Assignment Help
- All Assignment Help
- My Assignment Help
- Student Assignment Help
- Instant Assignment Help
- Cheap Assignment Help
- Global Assignment Help
- Write My Assignment
- Do My Assignment
- Solve My Assignment
- Make My Assignment
- Pay for Assignment Help
-
Management
- Financial Management Assignment Help
- Business Management Assignment Help
- Management Assignment Help
- Project Management Assignment Help
- Supply Chain Management Assignment Help
- Operations Management Assignment Help
- Risk Management Assignment Help
- Strategic Management Assignment Help
- Logistics Management Assignment Help
- Global Business Strategy Assignment Help
- Consumer Behavior Assignment Help
- MBA Assignment Help
- Portfolio Management Assignment Help
- Change Management Assignment Help
- Hospitality Management Assignment Help
- Healthcare Management Assignment Help
- Investment Management Assignment Help
- Market Analysis Assignment Help
- Corporate Strategy Assignment Help
- Conflict Management Assignment Help
- Marketing Management Assignment Help
- Strategic Marketing Assignment Help
- CRM Assignment Help
- Marketing Research Assignment Help
- Human Resource Assignment Help
- Business Assignment Help
- Business Development Assignment Help
- Business Statistics Assignment Help
- Business Ethics Assignment Help
- 4p of Marketing Assignment Help
- Pricing Strategy Assignment Help
- Nursing
-
Finance
- Finance Assignment Help
- Do My Finance Assignment For Me
- Financial Accounting Assignment Help
- Behavioral Finance Assignment Help
- Finance Planning Assignment Help
- Personal Finance Assignment Help
- Financial Services Assignment Help
- Forex Assignment Help
- Financial Statement Analysis Assignment Help
- Capital Budgeting Assignment Help
- Financial Reporting Assignment Help
- International Finance Assignment Help
- Business Finance Assignment Help
- Corporate Finance Assignment Help
-
Accounting
- Accounting Assignment Help
- Managerial Accounting Assignment Help
- Taxation Accounting Assignment Help
- Perdisco Assignment Help
- Solve My Accounting Paper
- Business Accounting Assignment Help
- Cost Accounting Assignment Help
- Taxation Assignment Help
- Activity Based Accounting Assignment Help
- Tax Accounting Assignment Help
- Financial Accounting Theory Assignment Help
-
Computer Science and IT
- Robotics Assignment Help
- Operating System Assignment Help
- Data mining Assignment Help
- Computer Network Assignment Help
- Database Assignment Help
- IT Management Assignment Help
- Network Topology Assignment Help
- Data Structure Assignment Help
- Business Intelligence Assignment Help
- Data Flow Diagram Assignment Help
- UML Diagram Assignment Help
- R Studio Assignment Help
-
Law
- Law Assignment Help
- Business Law Assignment Help
- Contract Law Assignment Help
- Tort Law Assignment Help
- Social Media Law Assignment Help
- Criminal Law Assignment Help
- Employment Law Assignment Help
- Taxation Law Assignment Help
- Commercial Law Assignment Help
- Constitutional Law Assignment Help
- Corporate Governance Law Assignment Help
- Environmental Law Assignment Help
- Criminology Assignment Help
- Company Law Assignment Help
- Human Rights Law Assignment Help
- Evidence Law Assignment Help
- Administrative Law Assignment Help
- Enterprise Law Assignment Help
- Migration Law Assignment Help
- Communication Law Assignment Help
- Law and Ethics Assignment Help
- Consumer Law Assignment Help
- Science
- Biology
- Engineering
-
Humanities
- Humanities Assignment Help
- Sociology Assignment Help
- Philosophy Assignment Help
- English Assignment Help
- Geography Assignment Help
- Agroecology Assignment Help
- Psychology Assignment Help
- Social Science Assignment Help
- Public Relations Assignment Help
- Political Science Assignment Help
- Mass Communication Assignment Help
- History Assignment Help
- Cookery Assignment Help
- Auditing
- Mathematics
-
Economics
- Economics Assignment Help
- Managerial Economics Assignment Help
- Econometrics Assignment Help
- Microeconomics Assignment Help
- Business Economics Assignment Help
- Marketing Plan Assignment Help
- Demand Supply Assignment Help
- Comparative Analysis Assignment Help
- Health Economics Assignment Help
- Macroeconomics Assignment Help
- Political Economics Assignment Help
- International Economics Assignments Help
-
Academic Writing Services
-
Essay Writing
- Essay Help
- Essay Writing Help
- Essay Help Online
- Online Custom Essay Help
- Descriptive Essay Help
- Help With MBA Essays
- Essay Writing Service
- Essay Writer For Australia
- Essay Outline Help
- illustration Essay Help
- Response Essay Writing Help
- Professional Essay Writers
- Custom Essay Help
- English Essay Writing Help
- Essay Homework Help
- Literature Essay Help
- Scholarship Essay Help
- Research Essay Help
- History Essay Help
- MBA Essay Help
- Plagiarism Free Essays
- Writing Essay Papers
- Write My Essay Help
- Need Help Writing Essay
- Help Writing Scholarship Essay
- Help Writing a Narrative Essay
- Best Essay Writing Service Canada
-
Dissertation
- Biology Dissertation Help
- Academic Dissertation Help
- Nursing Dissertation Help
- Dissertation Help Online
- MATLAB Dissertation Help
- Doctoral Dissertation Help
- Geography Dissertation Help
- Architecture Dissertation Help
- Statistics Dissertation Help
- Sociology Dissertation Help
- English Dissertation Help
- Law Dissertation Help
- Dissertation Proofreading Services
- Cheap Dissertation Help
- Dissertation Writing Help
- Marketing Dissertation Help
- Programming
-
Case Study
- Write Case Study For Me
- Business Law Case Study Help
- Civil Law Case Study Help
- Marketing Case Study Help
- Nursing Case Study Help
- Case Study Writing Services
- History Case Study help
- Amazon Case Study Help
- Apple Case Study Help
- Case Study Assignment Help
- ZARA Case Study Assignment Help
- IKEA Case Study Assignment Help
- Zappos Case Study Assignment Help
- Tesla Case Study Assignment Help
- Flipkart Case Study Assignment Help
- Contract Law Case Study Assignments Help
- Business Ethics Case Study Assignment Help
- Nike SWOT Analysis Case Study Assignment Help
- Coursework
- Thesis Writing
- CDR
- Research
-
Assignment Writing
-
Resources
- Referencing Guidelines
-
Universities
-
Australia
- Asia Pacific International College Assignment Help
- Macquarie University Assignment Help
- Rhodes College Assignment Help
- APIC University Assignment Help
- Torrens University Assignment Help
- Kaplan University Assignment Help
- Holmes University Assignment Help
- Griffith University Assignment Help
- VIT University Assignment Help
- CQ University Assignment Help
-
Australia
- Experts
- Free Sample
- Testimonial
DATA4000 Introduction to Business Analytics Case Study 1 Sample
Your Task
Complete Parts A to C below by the due date.
Consider the rubric at the end of the assignment for guidance on structure and content.
Assessment Description
• You are to read case studies provided and answer questions in relation to the content, analytics theory and potential analytics professionals required for solving the business problems at hand.
• Learning outcomes 1 and 2 are addressed.
Assessment Instructions
Part A: Case Study Analysis (700 words, 10 marks)
Instructions: Read the following two case studies. For each case study, briefly describe:
a) The industry to which analytics has been applied
b) A potential and meaningful business problem to be solved
c) The type of analytics used, and how it was used to address that potential and meaningful business problem
d) The main challenge(s) of using this type of analytics to achieve your business objective (from part b)
e) Recommendations regarding how to be assist stakeholders with adapting these applications for their business.
Part B: The Role of Analytics in Solving Business Problems (500 words, 8 marks)
Instructions: Describe two different types of analytics (from Workshop 1) and evaluate how each could be used as part of a solution to a business problem with reference to ONE real-world case study of your own choosing for one type of analytics and a SECOND real-world case study of your choosing for the second type of analytics.
You will need to conduct independent research and consult resources provided in the subject.
Part C: Developing and Sourcing Analytics Capabilities
Instructions: You are the Chief Analytics Officer for a large multinational corporation in the communications sector with operations that span India, China, the Philippines and Australia.
The organization is undergoing significant transformations; it is scaling back operations in existing low revenue segments and ramping up investments in next generation products and services - 5G, cloud computing and Software as a Service (SaaS).
The business is keen to develop its data and analytics capabilities. This includes using technology for product innovation and for developing a large contingent of knowledge workers.
To prepare management for these changes, you have been asked review Accenture’s report
(see link below) and publish a short report of your own that addresses the following key points:
1. How do we best ingrain analytics into the organisation’s decision-making processes?
2. How do we organize and coordinate analytics capabilities across the organization?
3. How should we source, train and deploy analytics talent?
The report is prepared for senior management and the board of directors. It must reflect the needs of your organization and the sector you operate in (communications).
Solution
Part A
1. Netflix Predictive Analytics: Journey to 220Mn+ subscribers
a) The case study covers how predictive analytics are being used in a variety of sectors, such as e-commerce, insurance, and customer support.
b) Reducing client turnover is a possible and significant business issue that can be resolved with predictive analytics. Businesses may experience a serious issue with customer churn, which is the rate at which customers leave doing business with a company. It might lead to revenue loss and reputational harm for the business. For assignment help, Businesses may enhance customer retention and eventually boost revenue by identifying customers who are at danger of leaving and taking proactive measures to keep them.
c) Customer demands were predicted using predictive analytics, which also helped to decrease churn, increase productivity, better allocate resources, and deliver tailored marketing messages. Customer data was analyzed using machine learning algorithms to spot trends and forecast future behavior. Predictive analytics, for instance, was used to inform customer service professionals to take proactive measures to keep clients who were at risk of leaving based on their behavior (Fouladirad et al., 2018).
d) Having access to high-quality data is the biggest obstacle to employing predictive analytics to accomplish the business goal of reducing customer turnover. In order to produce precise forecasts, predictive analytics needs reliable and pertinent data. The forecasts won't be correct if the data is unreliable, erroneous, or out of date. Additionally, firms may have trouble locating the proper talent to create and maintain the predictive models because predictive analytics needs substantial computational resources.
e) It is advised to start with a specific business problem in mind and find the data necessary to address it in order to help stakeholders with designing predictive analytics solutions for their organization. Making sure the data is correct and pertinent to the issue being addressed is crucial. In order to create and maintain the predictive models, businesses should also invest in the appropriate computational tools and recruit qualified data scientists. To guarantee that the predictive models continue to be accurate and useful, organizations should regularly assess their effectiveness and make any necessary adjustments (Ryu, 2013).
2. Coca-Cola vs. Pepsi: The Sweet Fight For Data-Driven Supremacy
a) The article focuses on the food and beverage industry, particularly the competition between Pepsi and Coca-Cola in terms of leveraging data and analytics to create new products and enhance corporate processes.
b) Keeping up with shifting consumer preferences and tastes is one of the potential commercial challenges for Coca-Cola and Pepsi. The difficulty lies in creating new beverages that satisfy the changing market needs and offer a tailored client experience. This issue calls for a cutting-edge strategy that can use analytics and data to spot patterns and customer behavior instantly.
c) Coca-Cola and Pepsi have both utilized various types of data analytics to help in the development of new products. The Freestyle beverage fountain from Coca-Cola gathers information on the most popular flavor combinations that can be used to crowdsource new product ideas. In order to track long-term consumer behavior and use it to personalize marketing campaigns and customer experiences, Coca-Cola has also integrated AI, ML, and real-time analytics. As an alternative, Pepsi has validated new product ideas by using AI-powered food analytics technologies like Trendscope and Tastewise to forecast consumer preferences. In order to spot new trends and forecast customer demand, these technologies comb through billions of social media posts, interactions with recipes, and menu items.
d) The accuracy and dependability of the data is one of the key difficulties when employing data analytics to create new goods. There is a chance that goods will be developed that may not be commercially successful since the data may not always accurately reflect actual consumer preferences and behavior. The demand for qualified data scientists and analysts who can decipher the data and offer useful insights for business decision-making is also great.
e) It is critical for stakeholders to understand the business issue they are attempting to solve before they can adapt data analytics applications for that issue. In order to gain insights into client behavior and preferences, they should identify the pertinent data sources and analytics technologies. In order to evaluate the data and offer actionable insights for business decision-making, stakeholders need also invest in qualified data scientists and analysts. In order to spur innovation and maintain an edge over the competition, firms should also be open to experimenting with new technology and methods.
Part B
By offering data-driven insights that can guide decision-making, analytics plays a significant role in resolving complicated business problems. This section will go over the two forms of analytics that can be utilized to solve business issues in many industries, namely descriptive analytics and predictive analytics.
Descriptive Analytics
A type of analytics called descriptive analytics includes looking at past data to understand past performance. It is frequently used to summarize and comprehend data, spot trends, and respond to the query "what happened?" Businesses can gain a better understanding of their historical performance and pinpoint opportunities for development by utilizing descriptive analytics.
Walmart is one example of a real-world case study that shows the application of descriptive analytics (Hwang et al., 2016). To optimize its inventory levels, cut expenses, and enhance the customer experience, Walmart employs descriptive analytics to examine customer purchasing trends and preferences. To find patterns in customer behavior, Walmart's data analysts examine sales information from both physical shops and online shopping platforms. By stocking up on items that are in high demand and reducing inventory levels of items that are not selling well, the corporation can better utilize the knowledge gained from these analysis (Dapi, 2012).
Predictive Analytics
Contrarily, predictive analytics is a subset of analytics that uses previous data to forecast future outcomes. When analyzing data, it is frequently used to spot trends and connections, predict the future, and provide a solution to the question, "What will happen?" Businesses can use predictive analytics to make data-driven decisions that foresee potential trends and business possibilities in the future.
The ride-hailing service Uber serves as one example of a real-world case study that demonstrates the application of predictive analytics (Chen et al.,2021). Uber employs predictive analytics to forecast rider supply and demand in real-time, enabling it to give drivers more precise arrival times and shorten wait times for riders. The business's data experts estimate the demand and supply trends for riders by analyzing data from a variety of sources, including historical ride data, weather forecasts, and event calendars. Uber can deploy its drivers more effectively thanks to the information gained from these analytics, which decreases rider wait times and enhances the entire riding experience (Batat, 2020).
Challenges
There are issues with using descriptive and predictive analytics, despite the fact that they both provide useful information for enterprises. The fact that descriptive analytics can only be used to analyze past data, which might not be a reliable indicator of future trends, is one of the key drawbacks of this approach. Furthermore, descriptive analytics ignores outside variables that can affect future performance. Using predictive analytics, however, presents a number of difficulties because it depends on precise and trustworthy data inputs. Predictive analytics may not produce accurate forecasts if the data is unreliable or incomplete.
Part C
Introduction
As Chief Analytics Officer for a large multinational corporation operating in the communications sector, I have been asked to review Accenture's report on building analytics-driven organizations and provide recommendations on how our company can develop its data and analytics capabilities.
Ingraining Analytics into Decision-making Processes
We must first create a data-driven culture before integrating analytics into our decision-making procedures. This entails encouraging the application of data and analytics at every level of the business, from the C-suite to the shop floor. By assembling cross-functional teams including data scientists, business analysts, and subject matter experts, we can do this. Together, these teams should pinpoint business issues that data and analytics may help to address and create solutions that are simple to comprehend and put into practice.
Investing in technology that facilitates data-driven decision making is another method to integrate analytics into our decision-making processes. Investments in big data platforms, data visualization tools, and predictive analytics software all fall under this category. By utilizing these tools, we can make greater use of the data we gather and offer data-based, actionable insights to decision-makers.
Organizing and Coordinating Analytics Capabilities
We must create a clear analytics strategy that supports our business objectives in order to organize and coordinate analytics skills across the organization. This strategy should lay out our plans for using data and analytics to accomplish our corporate goals as well as the precise skills we'll need to build to get there.
Additionally, we must clearly define the roles and duties of our analytics teams. This entails outlining the functions of domain experts, business analysts, and data scientists as well as developing a clear career path for each of these positions. By doing this, we can make sure that our analytics teams are functioning well and effectively, and that we are utilizing all of our analytical expertise to its fullest potential (Akter et al., 2016).
Sourcing, Training, and Deploying Analytics Talent
We must first have a clear talent strategy before we can find, train, and deploy analytical talent. This plan should detail the talents and expertise we specifically need, as well as the methods we'll use to find and develop the talent we need. We may achieve this through collaborating with colleges and training facilities, providing internships and apprenticeships, and funding staff development and training programmers.
Using our current employees as a resource for analytics talent is another option. Employees that are interested in data and analytics can be identified, and we can offer them the chances for training and growth they require to become proficient data analysts or data scientists. By doing this, we can utilize the knowledge and talents of our current personnel.
Enhancing Collaboration and Communication
Encourage collaboration and communication amongst divisions in order to successfully incorporate analytics into the organization's decision-making processes. Data silos and communication obstacles might make it difficult to adopt analytics solutions successfully. In order for teams to collaborate and solve business problems, it is critical to develop cross-functional communication and collaboration.
Investing in Training and Development
It is important to invest in the training and development of personnel if you want to create and coordinate analytics capabilities across your organization. This involves giving opportunities for upskilling and reskilling as well as strengthening data literacy abilities. Businesses may make sure that their staff members have the knowledge and abilities to use analytics tools and make data-driven decisions by investing in their training and development (Fadler & Legner, 2021).
Partnering with External Experts
Organizations can think about partnering with external experts to hasten the development of analytics capabilities. To acquire the most recent research and insights, this may entail working with academic institutions or employing outside experts. Organizations can benefit from a new viewpoint and access to cutting-edge analytics tools by collaborating with external experts.
Adopting a Continuous Learning attitude
Organizations must embrace a continuous learning attitude in order to effectively find, train, and deploy analytical talent. This entails supporting a culture of learning among staff members as well as their ongoing skill and knowledge development. In order to keep the company competitive in the market, it is also crucial to stay up to speed with the most recent analytics trends and technology. By adopting a continuous learning mindset, organizations can create a dynamic workforce that is agile and responsive to changing business needs.
Conclusion
To transform into an analytics-driven organization, we must create a data-driven culture, invest in the technology that enables data-driven decision-making, develop a clear analytics strategy, define the roles and responsibilities of our analytics teams, and create a talent strategy that enables us to efficiently find, develop, and deploy analytics talent. By doing this, we can make sure that we are maximizing the use of the data we gather and utilizing data and analytics to promote corporate growth and success in the communications industry.
References
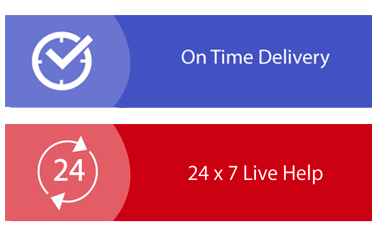
Download Samples PDF
Related Sample
- GAL613 Grief and Loss Assignment
- MBA642 Project Initiation, Planning and Execution Report 3
- MGT302A Strategic Management Assignment
- PROG2008 Computational Thinking Assignment 3
- BIS2005 Enterprise Architecture Assignment Report
- Business Decision Analytics Assessment
- CIS7030 Geospatial Analysis Assignment
- MK400 Agribusiness Marketing Report 3
- MGT613 Leadership For Sustainable Futures
- BSBWOR502B Ensure Team Effectiveness Assignment
- HLTWHS004 Manage work health and safety Assignment
- LAW1507 Tort Law Assignment
- CGRM4000 Corporate Governance Sustainability and Ethics Case Study
- BUS500 Business and Management Assignment
- MBA632 Knowledge Management
- STAT6200 Statistics for Public Health
- SHS7000 Food Safety in Association with Campylobacter in Chicken Report
- BSBRES801 Initiate and Lead Applied Research Assignment
- CPCCBC4012 Building and Construction Assignment
- HI6007 Statistics for Business Decisions Assignment Sample
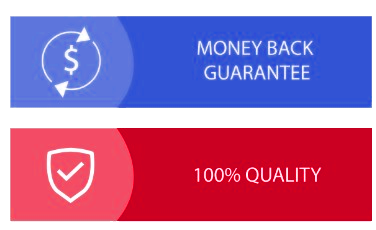
Assignment Services
-
Assignment Writing
-
Academic Writing Services
- Urgent Assignment Help
- Writing Assignment for University
- College Assignment Help
- SPSS Assignment Help
- HND Assignment Help
- Architecture Assignment Help
- Total Assignment Help
- All Assignment Help
- My Assignment Help
- Student Assignment Help
- Instant Assignment Help
- Cheap Assignment Help
- Global Assignment Help
- Write My Assignment
- Do My Assignment
- Solve My Assignment
- Make My Assignment
- Pay for Assignment Help
-
Management
- Financial Management Assignment Help
- Business Management Assignment Help
- Management Assignment Help
- Project Management Assignment Help
- Supply Chain Management Assignment Help
- Operations Management Assignment Help
- Risk Management Assignment Help
- Strategic Management Assignment Help
- Logistics Management Assignment Help
- Global Business Strategy Assignment Help
- Consumer Behavior Assignment Help
- MBA Assignment Help
- Portfolio Management Assignment Help
- Change Management Assignment Help
- Hospitality Management Assignment Help
- Healthcare Management Assignment Help
- Investment Management Assignment Help
- Market Analysis Assignment Help
- Corporate Strategy Assignment Help
- Conflict Management Assignment Help
- Marketing Management Assignment Help
- Strategic Marketing Assignment Help
- CRM Assignment Help
- Marketing Research Assignment Help
- Human Resource Assignment Help
- Business Assignment Help
- Business Development Assignment Help
- Business Statistics Assignment Help
- Business Ethics Assignment Help
- 4p of Marketing Assignment Help
- Pricing Strategy Assignment Help
- Nursing
-
Finance
- Finance Assignment Help
- Do My Finance Assignment For Me
- Financial Accounting Assignment Help
- Behavioral Finance Assignment Help
- Finance Planning Assignment Help
- Personal Finance Assignment Help
- Financial Services Assignment Help
- Forex Assignment Help
- Financial Statement Analysis Assignment Help
- Capital Budgeting Assignment Help
- Financial Reporting Assignment Help
- International Finance Assignment Help
- Business Finance Assignment Help
- Corporate Finance Assignment Help
-
Accounting
- Accounting Assignment Help
- Managerial Accounting Assignment Help
- Taxation Accounting Assignment Help
- Perdisco Assignment Help
- Solve My Accounting Paper
- Business Accounting Assignment Help
- Cost Accounting Assignment Help
- Taxation Assignment Help
- Activity Based Accounting Assignment Help
- Tax Accounting Assignment Help
- Financial Accounting Theory Assignment Help
-
Computer Science and IT
- Robotics Assignment Help
- Operating System Assignment Help
- Data mining Assignment Help
- Computer Network Assignment Help
- Database Assignment Help
- IT Management Assignment Help
- Network Topology Assignment Help
- Data Structure Assignment Help
- Business Intelligence Assignment Help
- Data Flow Diagram Assignment Help
- UML Diagram Assignment Help
- R Studio Assignment Help
-
Law
- Law Assignment Help
- Business Law Assignment Help
- Contract Law Assignment Help
- Tort Law Assignment Help
- Social Media Law Assignment Help
- Criminal Law Assignment Help
- Employment Law Assignment Help
- Taxation Law Assignment Help
- Commercial Law Assignment Help
- Constitutional Law Assignment Help
- Corporate Governance Law Assignment Help
- Environmental Law Assignment Help
- Criminology Assignment Help
- Company Law Assignment Help
- Human Rights Law Assignment Help
- Evidence Law Assignment Help
- Administrative Law Assignment Help
- Enterprise Law Assignment Help
- Migration Law Assignment Help
- Communication Law Assignment Help
- Law and Ethics Assignment Help
- Consumer Law Assignment Help
- Science
- Biology
- Engineering
-
Humanities
- Humanities Assignment Help
- Sociology Assignment Help
- Philosophy Assignment Help
- English Assignment Help
- Geography Assignment Help
- Agroecology Assignment Help
- Psychology Assignment Help
- Social Science Assignment Help
- Public Relations Assignment Help
- Political Science Assignment Help
- Mass Communication Assignment Help
- History Assignment Help
- Cookery Assignment Help
- Auditing
- Mathematics
-
Economics
- Economics Assignment Help
- Managerial Economics Assignment Help
- Econometrics Assignment Help
- Microeconomics Assignment Help
- Business Economics Assignment Help
- Marketing Plan Assignment Help
- Demand Supply Assignment Help
- Comparative Analysis Assignment Help
- Health Economics Assignment Help
- Macroeconomics Assignment Help
- Political Economics Assignment Help
- International Economics Assignments Help
-
Academic Writing Services
-
Essay Writing
- Essay Help
- Essay Writing Help
- Essay Help Online
- Online Custom Essay Help
- Descriptive Essay Help
- Help With MBA Essays
- Essay Writing Service
- Essay Writer For Australia
- Essay Outline Help
- illustration Essay Help
- Response Essay Writing Help
- Professional Essay Writers
- Custom Essay Help
- English Essay Writing Help
- Essay Homework Help
- Literature Essay Help
- Scholarship Essay Help
- Research Essay Help
- History Essay Help
- MBA Essay Help
- Plagiarism Free Essays
- Writing Essay Papers
- Write My Essay Help
- Need Help Writing Essay
- Help Writing Scholarship Essay
- Help Writing a Narrative Essay
- Best Essay Writing Service Canada
-
Dissertation
- Biology Dissertation Help
- Academic Dissertation Help
- Nursing Dissertation Help
- Dissertation Help Online
- MATLAB Dissertation Help
- Doctoral Dissertation Help
- Geography Dissertation Help
- Architecture Dissertation Help
- Statistics Dissertation Help
- Sociology Dissertation Help
- English Dissertation Help
- Law Dissertation Help
- Dissertation Proofreading Services
- Cheap Dissertation Help
- Dissertation Writing Help
- Marketing Dissertation Help
- Programming
-
Case Study
- Write Case Study For Me
- Business Law Case Study Help
- Civil Law Case Study Help
- Marketing Case Study Help
- Nursing Case Study Help
- Case Study Writing Services
- History Case Study help
- Amazon Case Study Help
- Apple Case Study Help
- Case Study Assignment Help
- ZARA Case Study Assignment Help
- IKEA Case Study Assignment Help
- Zappos Case Study Assignment Help
- Tesla Case Study Assignment Help
- Flipkart Case Study Assignment Help
- Contract Law Case Study Assignments Help
- Business Ethics Case Study Assignment Help
- Nike SWOT Analysis Case Study Assignment Help
- Coursework
- Thesis Writing
- CDR
- Research