Order Now
- Home
- About Us
-
Services
-
Assignment Writing
-
Academic Writing Services
- Urgent Assignment Help
- Writing Assignment for University
- College Assignment Help
- SPSS Assignment Help
- HND Assignment Help
- Architecture Assignment Help
- Total Assignment Help
- All Assignment Help
- My Assignment Help
- Student Assignment Help
- Instant Assignment Help
- Cheap Assignment Help
- Global Assignment Help
- Write My Assignment
- Do My Assignment
- Solve My Assignment
- Make My Assignment
- Pay for Assignment Help
-
Management
- Financial Management Assignment Help
- Business Management Assignment Help
- Management Assignment Help
- Project Management Assignment Help
- Supply Chain Management Assignment Help
- Operations Management Assignment Help
- Risk Management Assignment Help
- Strategic Management Assignment Help
- Logistics Management Assignment Help
- Global Business Strategy Assignment Help
- Consumer Behavior Assignment Help
- MBA Assignment Help
- Portfolio Management Assignment Help
- Change Management Assignment Help
- Hospitality Management Assignment Help
- Healthcare Management Assignment Help
- Investment Management Assignment Help
- Market Analysis Assignment Help
- Corporate Strategy Assignment Help
- Conflict Management Assignment Help
- Marketing Management Assignment Help
- Strategic Marketing Assignment Help
- CRM Assignment Help
- Marketing Research Assignment Help
- Human Resource Assignment Help
- Business Assignment Help
- Business Development Assignment Help
- Business Statistics Assignment Help
- Business Ethics Assignment Help
- 4p of Marketing Assignment Help
- Pricing Strategy Assignment Help
- Nursing
-
Finance
- Finance Assignment Help
- Do My Finance Assignment For Me
- Financial Accounting Assignment Help
- Behavioral Finance Assignment Help
- Finance Planning Assignment Help
- Personal Finance Assignment Help
- Financial Services Assignment Help
- Forex Assignment Help
- Financial Statement Analysis Assignment Help
- Capital Budgeting Assignment Help
- Financial Reporting Assignment Help
- International Finance Assignment Help
- Business Finance Assignment Help
- Corporate Finance Assignment Help
-
Accounting
- Accounting Assignment Help
- Managerial Accounting Assignment Help
- Taxation Accounting Assignment Help
- Perdisco Assignment Help
- Solve My Accounting Paper
- Business Accounting Assignment Help
- Cost Accounting Assignment Help
- Taxation Assignment Help
- Activity Based Accounting Assignment Help
- Tax Accounting Assignment Help
- Financial Accounting Theory Assignment Help
-
Computer Science and IT
- Robotics Assignment Help
- Operating System Assignment Help
- Data mining Assignment Help
- Computer Network Assignment Help
- Database Assignment Help
- IT Management Assignment Help
- Network Topology Assignment Help
- Data Structure Assignment Help
- Business Intelligence Assignment Help
- Data Flow Diagram Assignment Help
- UML Diagram Assignment Help
- R Studio Assignment Help
-
Law
- Law Assignment Help
- Business Law Assignment Help
- Contract Law Assignment Help
- Tort Law Assignment Help
- Social Media Law Assignment Help
- Criminal Law Assignment Help
- Employment Law Assignment Help
- Taxation Law Assignment Help
- Commercial Law Assignment Help
- Constitutional Law Assignment Help
- Corporate Governance Law Assignment Help
- Environmental Law Assignment Help
- Criminology Assignment Help
- Company Law Assignment Help
- Human Rights Law Assignment Help
- Evidence Law Assignment Help
- Administrative Law Assignment Help
- Enterprise Law Assignment Help
- Migration Law Assignment Help
- Communication Law Assignment Help
- Law and Ethics Assignment Help
- Consumer Law Assignment Help
- Science
- Biology
- Engineering
-
Humanities
- Humanities Assignment Help
- Sociology Assignment Help
- Philosophy Assignment Help
- English Assignment Help
- Geography Assignment Help
- Agroecology Assignment Help
- Psychology Assignment Help
- Social Science Assignment Help
- Public Relations Assignment Help
- Political Science Assignment Help
- Mass Communication Assignment Help
- History Assignment Help
- Cookery Assignment Help
- Auditing
- Mathematics
-
Economics
- Economics Assignment Help
- Managerial Economics Assignment Help
- Econometrics Assignment Help
- Microeconomics Assignment Help
- Business Economics Assignment Help
- Marketing Plan Assignment Help
- Demand Supply Assignment Help
- Comparative Analysis Assignment Help
- Health Economics Assignment Help
- Macroeconomics Assignment Help
- Political Economics Assignment Help
- International Economics Assignments Help
-
Academic Writing Services
-
Essay Writing
- Essay Help
- Essay Writing Help
- Essay Help Online
- Online Custom Essay Help
- Descriptive Essay Help
- Help With MBA Essays
- Essay Writing Service
- Essay Writer For Australia
- Essay Outline Help
- illustration Essay Help
- Response Essay Writing Help
- Professional Essay Writers
- Custom Essay Help
- English Essay Writing Help
- Essay Homework Help
- Literature Essay Help
- Scholarship Essay Help
- Research Essay Help
- History Essay Help
- MBA Essay Help
- Plagiarism Free Essays
- Writing Essay Papers
- Write My Essay Help
- Need Help Writing Essay
- Help Writing Scholarship Essay
- Help Writing a Narrative Essay
- Best Essay Writing Service Canada
-
Dissertation
- Biology Dissertation Help
- Academic Dissertation Help
- Nursing Dissertation Help
- Dissertation Help Online
- MATLAB Dissertation Help
- Doctoral Dissertation Help
- Geography Dissertation Help
- Architecture Dissertation Help
- Statistics Dissertation Help
- Sociology Dissertation Help
- English Dissertation Help
- Law Dissertation Help
- Dissertation Proofreading Services
- Cheap Dissertation Help
- Dissertation Writing Help
- Marketing Dissertation Help
- Programming
-
Case Study
- Write Case Study For Me
- Business Law Case Study Help
- Civil Law Case Study Help
- Marketing Case Study Help
- Nursing Case Study Help
- Case Study Writing Services
- History Case Study help
- Amazon Case Study Help
- Apple Case Study Help
- Case Study Assignment Help
- ZARA Case Study Assignment Help
- IKEA Case Study Assignment Help
- Zappos Case Study Assignment Help
- Tesla Case Study Assignment Help
- Flipkart Case Study Assignment Help
- Contract Law Case Study Assignments Help
- Business Ethics Case Study Assignment Help
- Nike SWOT Analysis Case Study Assignment Help
- Coursework
- Thesis Writing
- CDR
- Research
-
Assignment Writing
-
Resources
- Referencing Guidelines
-
Universities
-
Australia
- Asia Pacific International College Assignment Help
- Macquarie University Assignment Help
- Rhodes College Assignment Help
- APIC University Assignment Help
- Torrens University Assignment Help
- Kaplan University Assignment Help
- Holmes University Assignment Help
- Griffith University Assignment Help
- VIT University Assignment Help
- CQ University Assignment Help
-
Australia
- Experts
- Free Sample
- Testimonial
BDA60 Big Data and Analytics Sample
Case Study
Big Retail is an online retail shop in Adelaide, Australia. Its website, at which its users can explore different products and promotions and place orders, has more than 100,000 visitors per month. During checkout, each customer has three options: 1) to login to an existing account; 2) to create a new account if they have not already registered; or 3) to checkout as a guest. Customers’ account information is maintained by both the sales and marketing departments in their separate databases. The sales department maintains records of the transactions in their database. The information technology (IT) department maintains the website.
Every month, the marketing team releases a catalogue and promotions, which are made available on the website and emailed to the registered customers. The website is static; that is, all the customers see the same content, irrespective of their location, login status or purchase history.
Recently, Big Retail has experienced a significant slump in sales, despite its having a cost advantage over its competitors. A significant reduction in the number of visitors to the website and the conversion rate (i.e., the percentage of visitors who ultimately buy something) has also been observed. To regain its market share and increase its sales, the management team at Big Retail has decided to adopt a data-driven strategy. Specifically, the management team wants to use big data analytics to enable a customised customer experience through targeted campaigns, a recommender system and product association.
The first step in moving towards the data-driven approach is to establish a data pipeline. The essential purpose of the data pipeline is to ingest data from various sources, integrate the data and store the data in a ‘data lake’ that can be readily accessed by both the management team and the data scientists.
Task Instructions
Critically analyse the above case study and write a 1,500-word report. In your report, ensure that you:
• Identify the potential data sources that align with the objectives of the organisation’s data-driven strategy. You should consider both the internal and external data sources. For each data source identified, describe its characteristics. Make reasonable assumptions about the fields and format of the data for each of the sources;
• Identify the challenges that will arise in integrating the data from different sources and that must be resolved before the data are stored in the ‘data lake.’ Articulate the steps necessary to address these issues;
• Describe the ‘data lake’ that you designed to store the integrated data and make the data available for efficient retrieval by both the management team and data scientists. The system should be designed using a commercial and/or an open-source database, tools and frameworks. Demonstrate how the ‘data lake’ meets the big data storage and retrieval requirements.
• Provide a schematic of the overall data pipeline. The schematic should clearly depict the data sources, data integration steps, the components of the ‘data lake’ and the interactions among all the entities.
Solution
1 INTRODUCTION
Big Data and Analytics have become one the most important technology for the online marketplace. The online market is fully dependent upon the review and feedback of the customer who frequently visits the website. To gain more customers, the organization needs to analyze the overall data regarding the review, sales, profit, user rating etc. to the customers to attract them (Ahmed & Kapadia, 2017). For Assignment Help, Thus, data storing and analysing are important tasks in business intelligence. To conduct these tasks, the organization need to organize the data pipelining for the data effective data management by employing suitable design. In this paper, then big data and the underlying aspects will be discussed for Big Retail using the Data Lake Design and Pipelining (Lytvyn, Vysotska, Veres, Brodyak, & Oryshchyn, 2017).
2 CASE STUDY OVERVIEW
2.1 OVERVIEW OF ORGANIZATION
Big Retain is one of the online retails shops in Adelaide, Australia. It has a large number of products which can be explored by the customer by visiting the website. The organization has detected that they have in an average of 100000 visitors per month who visit and explore the products there. On that website, customers can find various products and they can purchase those by paying the amount. The organization uses to publish the updated catalogue of the products and mail that to the registered users and keep that available on the website. So, the customer can visit the website and can view the available products. They also make the price of the products reasonable compared to the competitors in the market to attract more customers. Their website is maintained by the Information Technology department of the organization.
2.2 PRESENT CONDITION AND PROBLEM
Big Retails has a good number of products that it uses to sell to customers at a reasonable price. However, in recent days, they have faced a big challenge for the significant reduction in the number of customers. They primarily suspect the non-maintenance or non-adoption of the data-driven strategy by which they should have visualized the purchase, sales and marketing scenario of the organization (Lv, Iqbal, & Chang, 2018). To overcome the problem, they have decided to adopt the data-driven strategy for the betterment of the future business. So, they are now interested in the application of Bid Data Analytics so that they can obtain a customised customer experience and the recommender system for attractive more customers towards their business.
3 BIG DATA AND ANALYTICS
3.1 POTENTIAL DATA SOURCES
Big Retails had maintained their data in the server without which the data cannot be managed. As the number of customers was about 100000 per month, the transaction is expected to be huge in terms of website hit, website visit and product purchase. Those customers who purchase the products used to provide the review and rating on their website. So, apart from the business data like sales, profit, marketing etc. they need to maintain those reviews, ratings etc. data as well (Husamaldin & Saeed, 2019). Additionally, those data are also helpful in getting insight into the views and demands of customers regarding the products. So, the data like reviews, ratings etc. in addition to the data like sales, profit, marketing etc. will be required to be maintained and managed in the Bog Data Environment, As the big data environment can be managed for the particularization of the data sources, thus, the organization need to identify the data source (Batvia, 2017). Hence, the data sources for Big
Retail are as follows:
1. Data through Transaction: Big Retails can get the data from the transaction of the customers. It can be achievable concerning the purchase scenario and the website visit by the customers. When the customers will visit the website, and purchase some products, the data should be stored in the Big Data (Subudhi, Rout, & Ghosh, 2019).
2. Data for Customer Demand: When the customer will purchase some product, that product may satisfy or dissatisfy the customers. According to the satisfaction level, customers use to provide their product review and rating for the same products. This kind of data is essential for data analytics and to show the present demand of the products of the customer (Liang, Guo, & Shen, 2018).
3. Data through Machine: Apart from the two sources of data that are mentioned earlier. Another type of data comes from the system of the organizations. This kind of data may contain the historical records of the sales, profits or loss, marketing, campaigns etc.
3.2 CHALLENGES IN DATA INTEGRATION
Data Integration is a sensitive issue in Big Data Analytics. As Big Retail has a large volume of data and they wish to adopt big data analytics, they should be focused on the mitigation policies of the challenges that can be faced by then in the maintenance of the big data (Anandakumar, Arulmurugan, & Onn, 2019). Hence, there is a number of challenges that can be faced by Big Retail. The possible challenges of the Big Data Analytics that may be faced by Big Retails are as follows:
1. Data Quality: When Big Retail will adopt big data analytics for their business, the data should, be collected and stored in real-time by fetching those from the website. To control and maintain the huge volume of data, the quality of data places a significant impact. One of the greatest issues that can be generated during the maintenance of the data quality is the missing data (Anandakumar, Arulmurugan, & Onn, 2019). If the data contains missing values, the data will not be suitable for analytical work and so, the organization cannot operate of data. To get suitable data, the data sources and the data quality both need to be maintained.
2. Wrong Integration Process: The data integration process can connect the big data with the software ecosystem. A trigger-based data integration process allows the integration of the data with several applications that are aligned together. However, this process does not allow the integration of historical data which can be resolved by applying the Two-Way Integration System (Lytvyn, Vysotska, Veres, Brodyak, & Oryshchyn, 2017).
3. Data Overflow: Data should be collected by Big Retails based on the importance. If too much data will be collected regarding features, data can be overflown which is not expected for big data analytics.
3.3 DATA LAKE
A Data Lake can be defined by the repository of data that can accommodate a large amount of data of different formats such as structured data, semi-structured data and unstructured data. The greatest advantage of the application of data lake is that it allows the storage of the data without any limit. In this context, the data storage capacity is made flexible. It also facilitates the organization to store the data with high quality and with data integration (Liang, Guo, & Shen, 2018). These facilities increase the performance of data analytics on the big data which should be the expected scenario of Big Retail when they will adopt Big Data Analytics. Another advantage of the data lake is that it allows the storage of the data in real-time and while storing the data, the process is automated.
The data lake that can be proposed for Big Retail for making the business process smooth and faster is as follows:
Fig-1: Data Lake Design for Big Retail
3.4 DATA PIPELINE
The design of the data lake has been shown in the last section. The data pipelining can be addressed and demonstrated by emphasizing the data lake model for Big Retail. The process of data pipelining will follow the sequential operation of the data lake architecture (Ahmed & Kapadia, 2017). The data pipelining is discussed below:
1. Data Sources: Big Retail can gather the data by selecting the data source such as its website. Ads the data will be collected from the website, so the data may be the combined format of structured, unstructured or semi-structured.
2. Ingestion Tier: The data can be loaded in the data lake architecture in real-time or through batches as per the requirement (Lv, Iqbal, & Chang, 2018).
3. Unified Operations Tier: The data and the entire data management process will be controlled in this tier. it may also include the subordinate system that can manage the data, the workflow of the data collection and integration etc.
4. Processing Tier: After the data has been processed to the system of Big Retail, the analytics will be applied in this tier. This will facilitate the analysis process of the collected data so that the data insight can be generated (Batvia, 2017).
5. Distillation Tier: In the processing layer, the data of Big Retail will be analyzed using the employed algorithms. However, the processing time for the analytics is faster in the case of structured data. This tier is employed in the data lake to convert the collected unstructured and semi-structured data into structured one for faster analytics (Anandakumar, Arulmurugan, & Onn, 2019).
6. Insights Tier: The architecture of the data lake will employ the database queries on the data for the purpose of data analysis. It will help to compute the customer-based scenario such as sales per period, type of products with higher and lower sales etc.
7. Action: Finally, the architecture will produce visual insight into the data. In most cases, the visual insight may contain the analysis such as Review word cloud, Rating analysis, purchase statistics etc.
4 CONCLUSION
In this paper, big data analytics has been discussed for Big Retail through the implication of data lake and data pipelining. These measures have been seen to be effective in data management and analytics. As the number of customers is consistently decreasing for Big Retail, this architecture will help them grow their future business.
5 REFERENCES
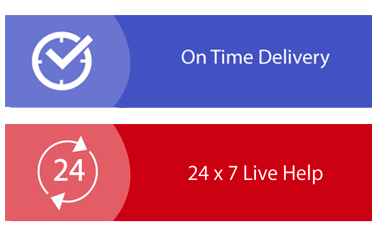
Download Samples PDF
Related Sample
- SIT763 Cyber Security Management Assignment
- The Australian Solicitors Conduct Rules
- MGT600 Team Performance Evaluation Assignment
- HCCSSD102 Person Centred Practice Report 2
- EBP107 Evidence Based Practice Assignment
- CAM529 Introduction To Public Health Assignment
- BUGEN5930 Business Society and The Planet Assignment
- MIS101 Information Systems for Business
- PUBH6006 Community Health and Disease Prevention
- Novel Protein Acherons Distribution Using Fluorescence Microscopy Assignment
- BUSM4738 Strategy Assignment
- Accounting Coursework Assignment
- MBA401 People, Culture and Contemporary Leadership Report 3
- MBA642 Project Initiation Planning and Execution Assignment
- NURBN1015 Introduction to Evidence Based Practice and Research Assignment
- Political Decision Making Process Affecting Global Leadership in International Business
- PROJ6000 Principles of Project Management Report
- MITS5002 Software Engineering Methodology Assignment
- 5011SP5 Accounting for Management M Report
- ACCT6004 Management Accounting Assignment
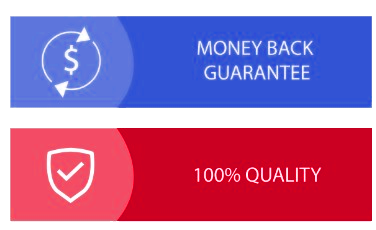
Assignment Services
-
Assignment Writing
-
Academic Writing Services
- Urgent Assignment Help
- Writing Assignment for University
- College Assignment Help
- SPSS Assignment Help
- HND Assignment Help
- Architecture Assignment Help
- Total Assignment Help
- All Assignment Help
- My Assignment Help
- Student Assignment Help
- Instant Assignment Help
- Cheap Assignment Help
- Global Assignment Help
- Write My Assignment
- Do My Assignment
- Solve My Assignment
- Make My Assignment
- Pay for Assignment Help
-
Management
- Financial Management Assignment Help
- Business Management Assignment Help
- Management Assignment Help
- Project Management Assignment Help
- Supply Chain Management Assignment Help
- Operations Management Assignment Help
- Risk Management Assignment Help
- Strategic Management Assignment Help
- Logistics Management Assignment Help
- Global Business Strategy Assignment Help
- Consumer Behavior Assignment Help
- MBA Assignment Help
- Portfolio Management Assignment Help
- Change Management Assignment Help
- Hospitality Management Assignment Help
- Healthcare Management Assignment Help
- Investment Management Assignment Help
- Market Analysis Assignment Help
- Corporate Strategy Assignment Help
- Conflict Management Assignment Help
- Marketing Management Assignment Help
- Strategic Marketing Assignment Help
- CRM Assignment Help
- Marketing Research Assignment Help
- Human Resource Assignment Help
- Business Assignment Help
- Business Development Assignment Help
- Business Statistics Assignment Help
- Business Ethics Assignment Help
- 4p of Marketing Assignment Help
- Pricing Strategy Assignment Help
- Nursing
-
Finance
- Finance Assignment Help
- Do My Finance Assignment For Me
- Financial Accounting Assignment Help
- Behavioral Finance Assignment Help
- Finance Planning Assignment Help
- Personal Finance Assignment Help
- Financial Services Assignment Help
- Forex Assignment Help
- Financial Statement Analysis Assignment Help
- Capital Budgeting Assignment Help
- Financial Reporting Assignment Help
- International Finance Assignment Help
- Business Finance Assignment Help
- Corporate Finance Assignment Help
-
Accounting
- Accounting Assignment Help
- Managerial Accounting Assignment Help
- Taxation Accounting Assignment Help
- Perdisco Assignment Help
- Solve My Accounting Paper
- Business Accounting Assignment Help
- Cost Accounting Assignment Help
- Taxation Assignment Help
- Activity Based Accounting Assignment Help
- Tax Accounting Assignment Help
- Financial Accounting Theory Assignment Help
-
Computer Science and IT
- Robotics Assignment Help
- Operating System Assignment Help
- Data mining Assignment Help
- Computer Network Assignment Help
- Database Assignment Help
- IT Management Assignment Help
- Network Topology Assignment Help
- Data Structure Assignment Help
- Business Intelligence Assignment Help
- Data Flow Diagram Assignment Help
- UML Diagram Assignment Help
- R Studio Assignment Help
-
Law
- Law Assignment Help
- Business Law Assignment Help
- Contract Law Assignment Help
- Tort Law Assignment Help
- Social Media Law Assignment Help
- Criminal Law Assignment Help
- Employment Law Assignment Help
- Taxation Law Assignment Help
- Commercial Law Assignment Help
- Constitutional Law Assignment Help
- Corporate Governance Law Assignment Help
- Environmental Law Assignment Help
- Criminology Assignment Help
- Company Law Assignment Help
- Human Rights Law Assignment Help
- Evidence Law Assignment Help
- Administrative Law Assignment Help
- Enterprise Law Assignment Help
- Migration Law Assignment Help
- Communication Law Assignment Help
- Law and Ethics Assignment Help
- Consumer Law Assignment Help
- Science
- Biology
- Engineering
-
Humanities
- Humanities Assignment Help
- Sociology Assignment Help
- Philosophy Assignment Help
- English Assignment Help
- Geography Assignment Help
- Agroecology Assignment Help
- Psychology Assignment Help
- Social Science Assignment Help
- Public Relations Assignment Help
- Political Science Assignment Help
- Mass Communication Assignment Help
- History Assignment Help
- Cookery Assignment Help
- Auditing
- Mathematics
-
Economics
- Economics Assignment Help
- Managerial Economics Assignment Help
- Econometrics Assignment Help
- Microeconomics Assignment Help
- Business Economics Assignment Help
- Marketing Plan Assignment Help
- Demand Supply Assignment Help
- Comparative Analysis Assignment Help
- Health Economics Assignment Help
- Macroeconomics Assignment Help
- Political Economics Assignment Help
- International Economics Assignments Help
-
Academic Writing Services
-
Essay Writing
- Essay Help
- Essay Writing Help
- Essay Help Online
- Online Custom Essay Help
- Descriptive Essay Help
- Help With MBA Essays
- Essay Writing Service
- Essay Writer For Australia
- Essay Outline Help
- illustration Essay Help
- Response Essay Writing Help
- Professional Essay Writers
- Custom Essay Help
- English Essay Writing Help
- Essay Homework Help
- Literature Essay Help
- Scholarship Essay Help
- Research Essay Help
- History Essay Help
- MBA Essay Help
- Plagiarism Free Essays
- Writing Essay Papers
- Write My Essay Help
- Need Help Writing Essay
- Help Writing Scholarship Essay
- Help Writing a Narrative Essay
- Best Essay Writing Service Canada
-
Dissertation
- Biology Dissertation Help
- Academic Dissertation Help
- Nursing Dissertation Help
- Dissertation Help Online
- MATLAB Dissertation Help
- Doctoral Dissertation Help
- Geography Dissertation Help
- Architecture Dissertation Help
- Statistics Dissertation Help
- Sociology Dissertation Help
- English Dissertation Help
- Law Dissertation Help
- Dissertation Proofreading Services
- Cheap Dissertation Help
- Dissertation Writing Help
- Marketing Dissertation Help
- Programming
-
Case Study
- Write Case Study For Me
- Business Law Case Study Help
- Civil Law Case Study Help
- Marketing Case Study Help
- Nursing Case Study Help
- Case Study Writing Services
- History Case Study help
- Amazon Case Study Help
- Apple Case Study Help
- Case Study Assignment Help
- ZARA Case Study Assignment Help
- IKEA Case Study Assignment Help
- Zappos Case Study Assignment Help
- Tesla Case Study Assignment Help
- Flipkart Case Study Assignment Help
- Contract Law Case Study Assignments Help
- Business Ethics Case Study Assignment Help
- Nike SWOT Analysis Case Study Assignment Help
- Coursework
- Thesis Writing
- CDR
- Research